Agentic AI
BLOG
11 min read
Agentic AI in Automation: The Next Generation of Autonomous Intelligence
Just give it a thought: Your business has an AI that not only follows your command but also takes the initiative to act. You heard it right. Agentic AI is a new revolution in automation where systems not only generate responses but also take a step further to achieve goals.
The buzz for the term "Agentic AI" is on the rise, as McKinsey states that 40% of companies are either incorporating or planning to incorporate AI into automation methods.
When ChatGPT entered the scene, enterprises from different industries started to integrate Generative AI within their processes across functions like image generation, customer service, billing invoices and more.
According to a Google Cloud study, 70% of companies saw an increase in ROI after its adoption.
Agentic AI promises to adapt and respond to queries autonomously with minimal human intervention. This transformative approach to automation allows organizations to achieve high efficiency and innovation. Compared to traditional AI, which primarily depends on predefined commands, Agentic AI is about making real-time decisions based on environmental changes and organizational goals.
Looking to learn more about it?
Talk to Our Experts Today!Agentic AI has evolved more than generative functions, making processes smooth, fast and responsive. By 2025, the Global AI market will reach $3.9 trillion. Companies in sectors like healthcare and finance are taking notice of Agentic AI due to its capability of streamlining and optimizing decision-making. It is setting a new standard for enterprise innovation.
This article will introduce the primary concept of Agentic AI and showcase its rapid adoption in different business sectors. Key takeaways from this blog will be the impact of Agentic AI and its future implications for the business landscape.
What is Agentic AI? The New Wave in Automation
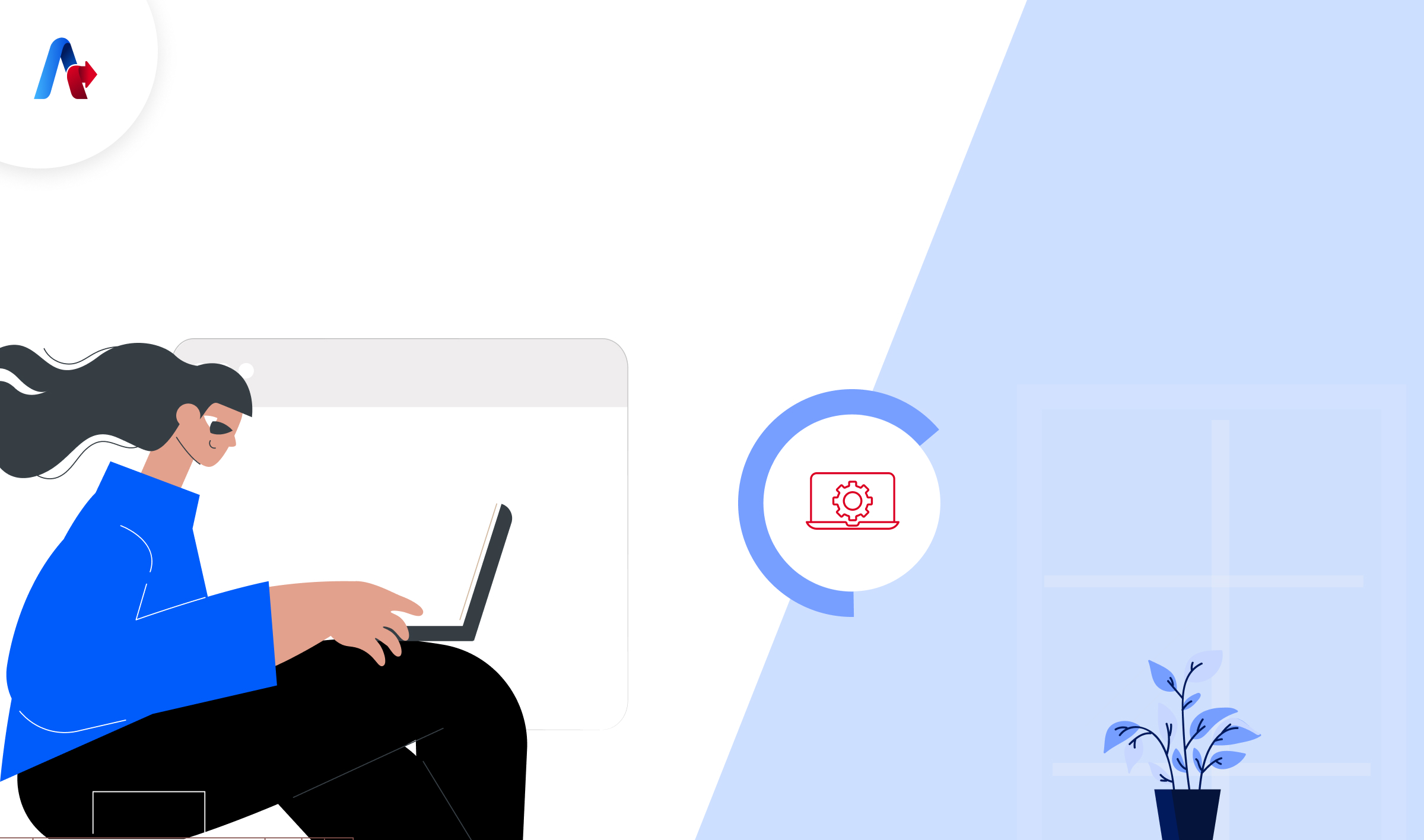
Agentic AI is a type of artificial intelligence that can function autonomously by using advanced algorithms and facilitating data-driven decision-making. Traditional AI requires human guidance, while Agentic AI approaches use machine learning and natural language processing to analyze data, recognize patterns and make choices on their own.
These AI systems incorporate different technologies, like neural networks and reinforcement learning, to constantly monitor their performance. The concept of IT automation has been transformed due to services like self-monitoring, self-healing and auto-scaling Technologies like Kubernetes, Docker and Terraform. These Technologies are vastly concerned with the principles of cybernetics self-action, which falls under the umbrella of Agentic AI.
Agentic AI does not require detailed coding from engineers. It allows anyone to interact using natural language, making it easier for non-technical users. It mainly utilizes large language models (LLMs) to create code while responsive to unique situations without static scripts. Agentic AI can analyze tasks, update progress, and formulate the following activities like human intervention.
The Evolution of Agentic AI Systems
It's important to understand what Agentic means and how it has evolved with time. Agentic AI is not just another development in automation and artificial intelligence: It's a transformation. Historically, automation was mostly dependent on Rule-Based Systems, where a set of stated rules dictated the entire behavior of systems. The emergence of machine learning facilitated models' learning from data and ensured the creation of a supervised learning technique that involved labeled data sets to train.
After that, deep learning was introduced, which used neural networks to analyze vast amounts of unstructured data. The involvement of several architectures, including Convolutional Neural Networks and Recurrent Neural Networks, made machines more intelligent in analyzing and interpreting complex data like images and natural language.
Then, in 2020, large language models like GPT-3 were involved, marking the primary steps of Agentic AI in understanding human text and context. It tackled the problem of extensive coding for running an automated system. The use of Transformer architectures allowed the concept of self-attention to function.
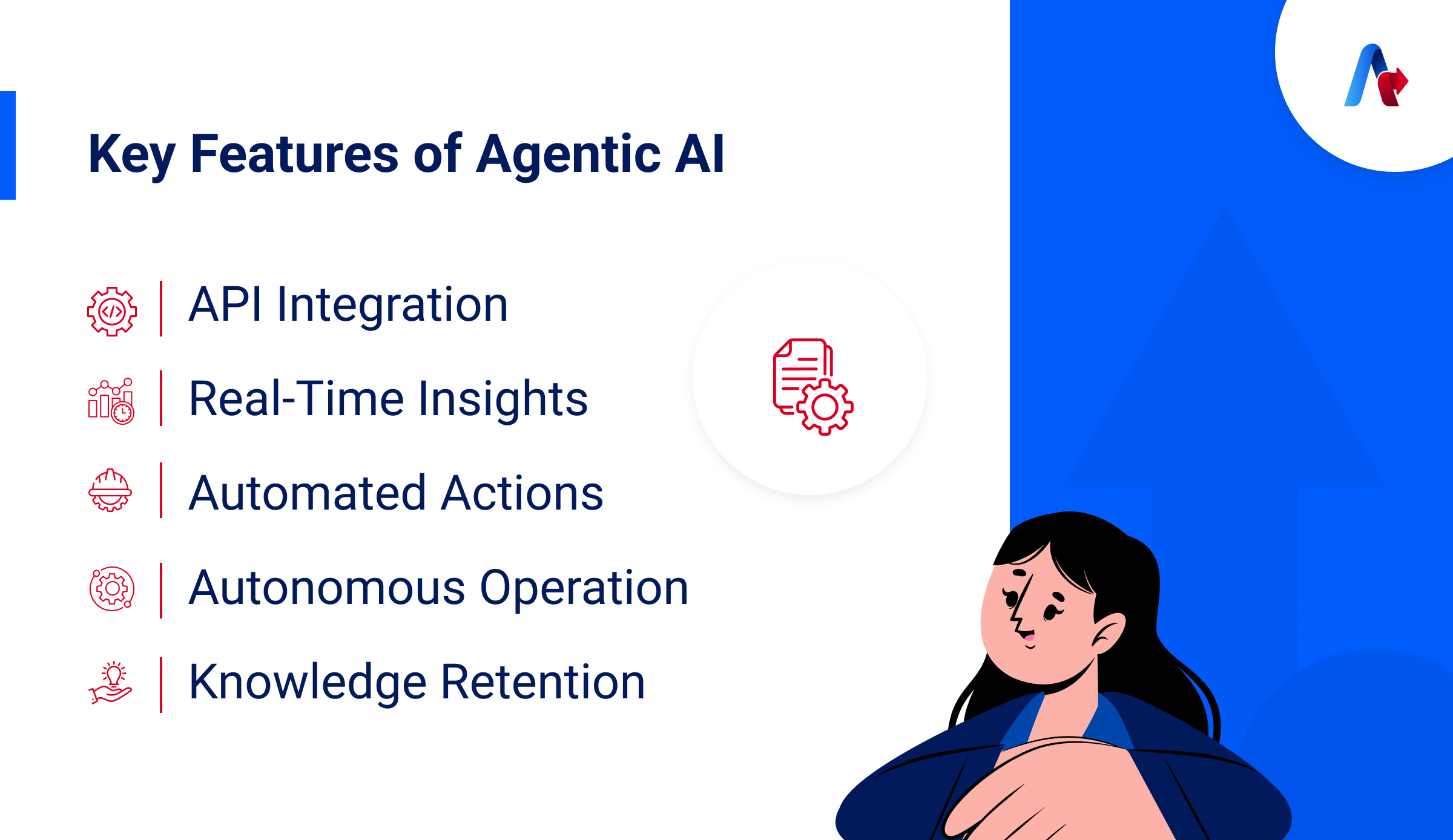
Agentic AI indicates Reinforcement Learning, which allows systems to optimize results based on feedback loops. AI agents create strategies depending on the outcomes, enhancing real-time decision-making attributes.
Gartner has highlighted in its reports that, by 2025, 75% of organizations will be using some type of Agentic AI, which specifies its future significance in business operations.
Studies have further indicated that companies adopting this system can generate a 30% deduction from their operational expenses by automating routine tasks and enhancing their decision-making capabilities.
- Tool Calling: Agentic AI can access external API and information to make proper decisions.
- Discovery: It can gather real-time data from different sources, creating a place for optimized analysis.
- Execution: It can perform actions like sending emails and processing transactions without the requirement of human oversight.
- Autonomy: These systems can operate effectively due to self-prompting functions, making it easier to accomplish the goals.
- Memory: It can retain knowledge from past actions, highlighting better decision-making in the future.
What is Agentic Process Automation?
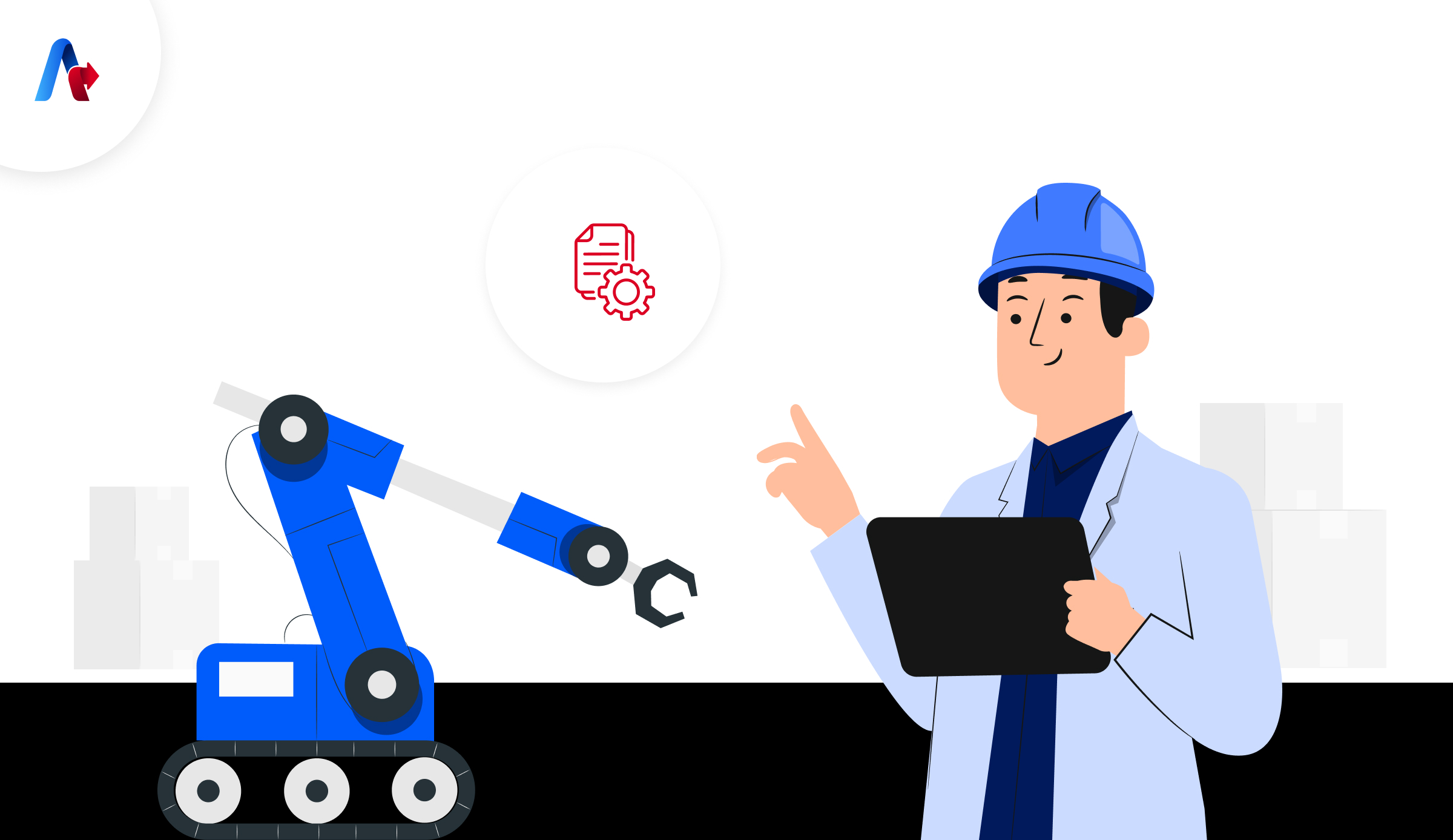
Agentic Process Automation (APA) has transformed conventional automation by incorporating advanced AI features that allow systems to work autonomously. Conventional automation relies on predefined scripts, while APA can use machine learning and natural language processing to solve problems and make real-time decisions.
APA's use of LLMs enhances responsiveness to interpret complex queries and take effective actions. This allows non-technical users to interact with systems and get their work done. They employ reinforcement learning techniques, which help them learn from historical data and adapt processes according to feedback loops, creating an effective chain of optimization.
APA can access and utilize APIs autonomously and execute transactions without human intervention. For example, an Agentic system can predict demand fluctuations and communicate with suppliers in supply chain management. Its design facilitates self-prompting while continuously checking the environment to act at the right moment. Agentic process automation is another step towards fully autonomous systems that optimize complex organization mechanisms with minimum human intervention.
How Agentic AI Differs from Traditional AI and RPA?
In this section, we have created a simplified table outlining the differences between Sales Cloud and Service Cloud based on some major distinguishing factors.
Feature | Agentic AI | Traditional AI | RPA |
---|---|---|---|
Operational Efficiency | Reduction of 50% in bottlenecks. | It varies, mostly less than 30%. | Reduces expenses by 30%. |
Cost Reduction | The ability to adjust increases financial performance. | Static cost savings. | Cost savings as per fixed methods. |
Scalability | Provides an increase of 60% in scalability. | Limited scalable options. | According to predefined workflows. |
Agentic AI gives automation an upper hand by emphasizing a level of autonomy that is missing from Conventional AI and Robotic Process Automation. Conventional AI is dependent on structured information and predefined algorithms, while Agentic AI uses LLMs that can easily analyze and structure data and respond in natural language.
RPA mainly automates tasks within defined workflows that demand structured scripting and human intervention. There is always a debate to choose between the two, Agentic AI and Generative AI, due to their functions. With Agentic AI having the potential to capable of making choices, Generative AI can manage content creation, based on human input.
According to Gartner, RPA can minimize operational expenses by 30%; however, it still functions within the challenges of pre-configured scripts. On the other hand, Agentic AI can generate responses according to changing conditions in real-time, emphasizing efficiency and decision-making capabilities more.
RPA needs human oversight and extensive coding to set up and manage. Agentic AI has a self-prompting mechanism that allows systems to access, plan and execute activities autonomously. The incorporation of real-time data and external APIs allows Agentic AI to optimize processes, making it easier for businesses to compete and sustain in this fast-paced environment.
Advantages and Disadvantages of Agentic AI
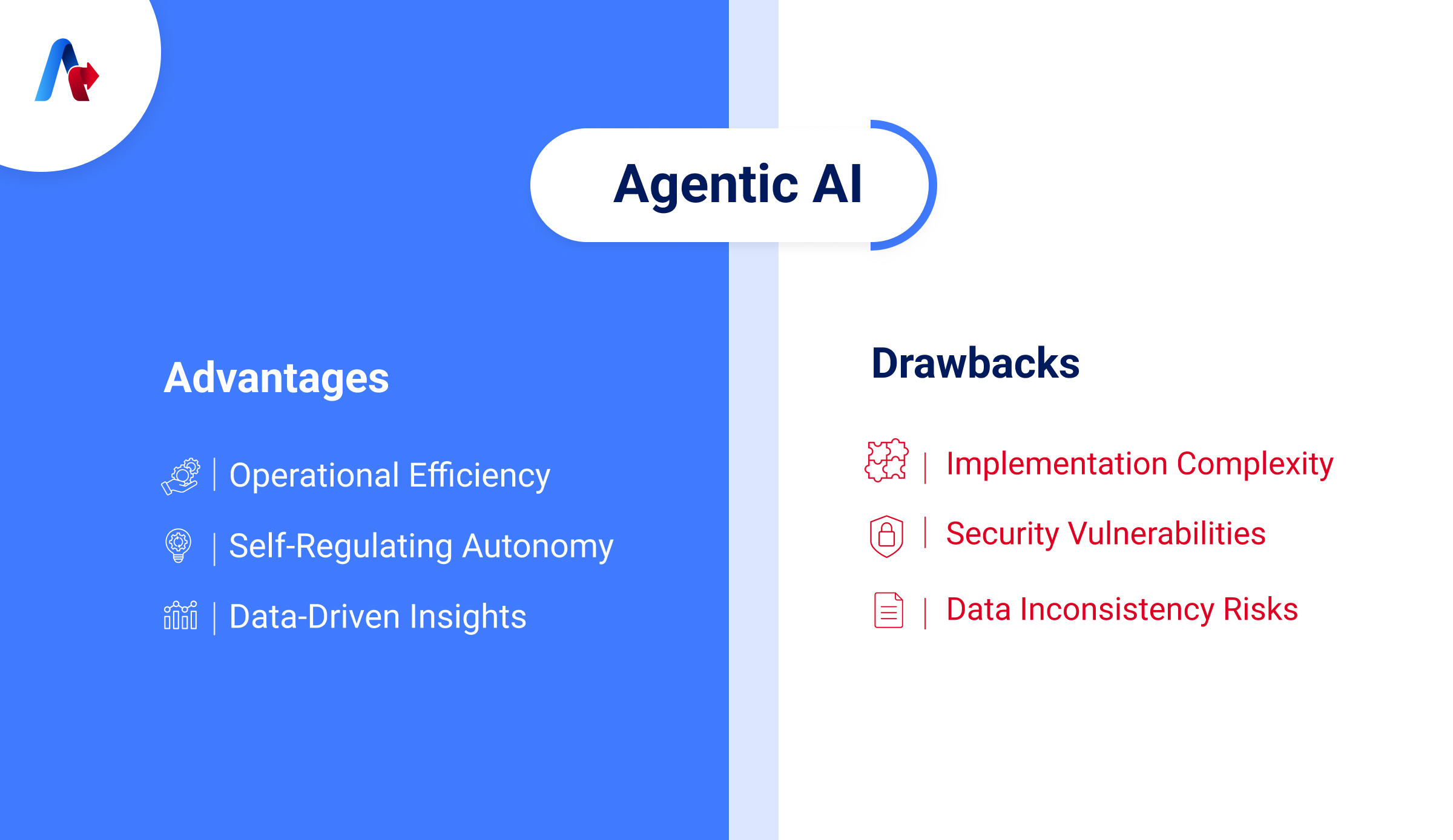
Agentic AI is changing the way we think about and use automation. It is taking automation a notch higher by allowing systems to operate independently and make decisions on their own. This technology can make work more efficient, reduce delays, and offer valuable insights based on data. However, adopting Agentic AI also comes with some challenges, like setup complexity, security concerns, and the need for accurate data. That’s where an industry expert like Accelirate can help you create a clear roadmap. Here is a simple breakdown of the benefits and drawbacks of using Agentic AI in business.
Advantages
- Operational Efficiency: Agentic AI can streamline complicated workflows through intelligent automation, minimize latency and increase output. Its ability to analyze and optimize task execution creates an adequate space for resource allocation and operational performance.
- Self-Regulating Autonomy: Features like self-prompting and adaptive learning enable Agentic AI to monitor systems and take necessary actions autonomously without any human intervention. This minimizes operational oversight and accelerates responsiveness, enabling rapid scaling.
- Data-Driven Insights: Employing advanced machine learning algorithms enables these processes to cover large data sets and formulate actionable insights. Its predictive analytics ability helps enterprises make informed choices, specifically in the areas of strategy formulation and risk management.
Disadvantages
- Complexity in Implementation: When setting up an Agentic AI system, it is necessary to integrate it with legacy systems. This requires high investments in infrastructure and labor. During the early phases, disruptions in the organization might occur while adapting the process.
- Security Limitations: The autonomous characteristic of agentic AI systems raises security concerns. Without the prevalence of high-end security frameworks, the systems are vulnerable to attacks, which can cause outcomes like system manipulation and data breaches.
- Inconsistency: The efficiency of agentic AI is based on the accuracy and integrity of data. If the data inputs are inaccurate, it might compromise the reliability of office results, creating flawed decision-making and problems in operation.
Want to integrate Agentic AI into your processes?
Let’s Talk About Your NeedsUnderstanding the Implications of Agentic AI for Organizations
We will explore three sectors where Agentic AI is making strides, emphasizing dynamic automation and intelligent decision-making in real-time. Particularly, the Agentic AI use cases will be studied, to understand how it is being used in several industries.
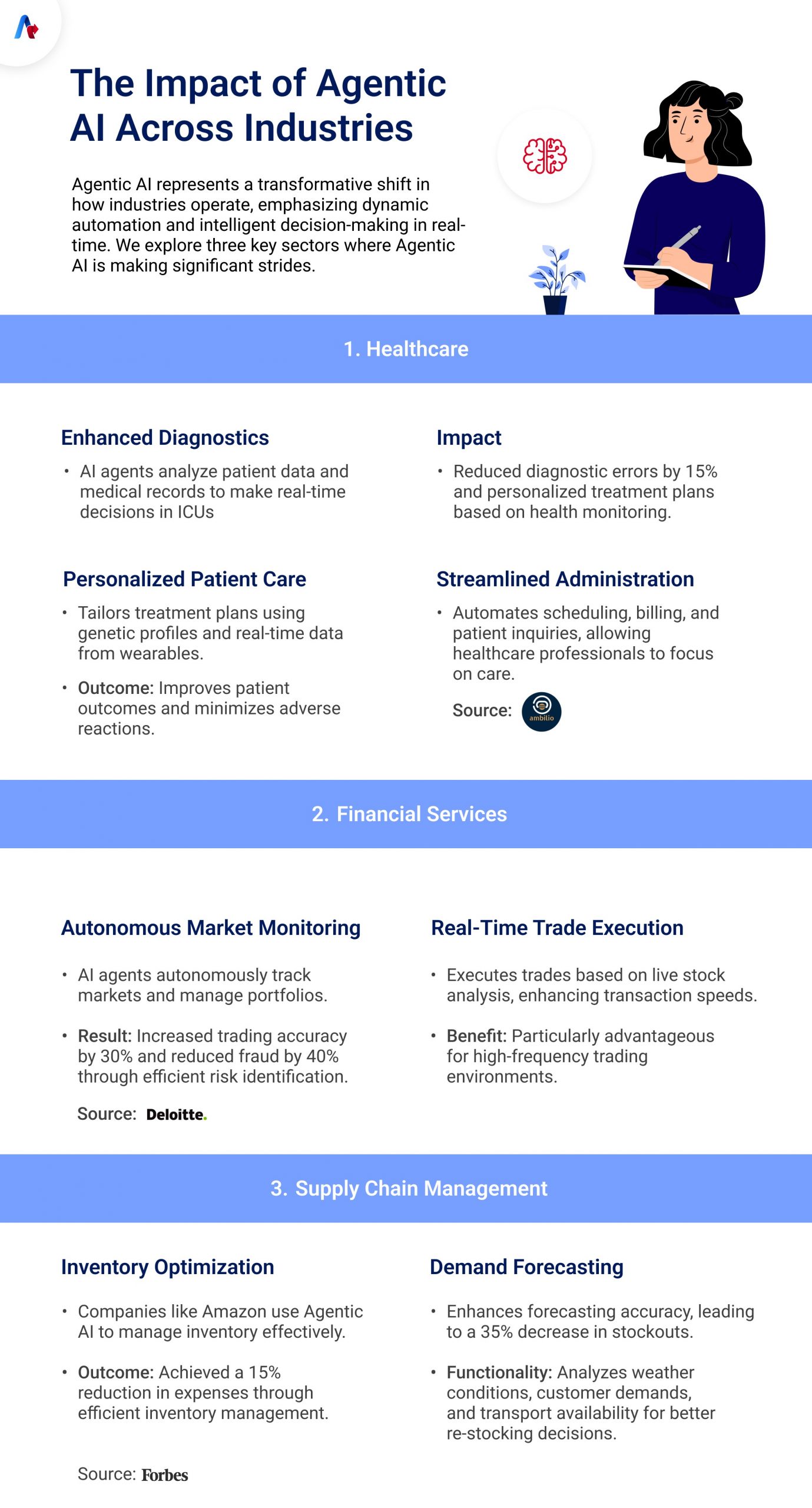
Healthcare
Agentic AI is becoming a domestic name within the healthcare sector, specifically in diagnostic and patient care. For example, AI agents are utilized to analyze and process patient data and medical records and make real-time decisions in ICUs. This approach has minimized diagnostic errors by 15% in specific systems and allows for a more customized patient treatment plan based on health monitoring (Source: McKinsey's).
Financial Services
Within finance, AI agents are helping to track and monitor markets and manage portfolios autonomously. The systems can execute trades based on real-time stock analysis. The use of these systems has shown an increase of 30% in trading accuracy by limiting human mistakes and maximizing the speeds of transactions. It has been highly beneficial for high-frequency trading. Certain banks have reported A reduction of 40% in frauds due to the use of agentic AI systems that identify risks efficiently (Source: Deloitte).
Supply Chain Management
The manufacturing and logistics sectors continually use AI agents to enhance operations and manage inventory. Amazon has initiated the use of Agentic AI systems to manage its inventory, leading to a 15% decrease in expenses. Other companies are implementing the systems in demand forecasting, resulting in a 35% decrease in stock outs. Their autonomous capabilities are a great help in re-stocking and re-routing as they can analyze weather conditions, customer demands and the availability of transport (Source: Forbes).
These Agentic AI examples create a clearer picture regarding its use, applications and real-life impact over several processes, Mostly, the caliber of generative AI has been exceeded with the introduction of Agentic AI enhancing the digital transformation at a rapid pace.
The Future of Agentic AI
The future of Agentic AI is exciting and holds transformative potential. It will develop on the parameters of autonomy and advanced interactivity within machine-driven processes. The system will evolve by incorporating simple language processing where agents can independently predict, plan, and execute complex activities. Using LLM-driven " code-on-demand" and real-time flexibility would enable the systems to transform domains like predictive maintenance, logistics and customized consumer experiences, leading to 60% operating profit. UiPath has developed an agent builder tool within the UiPath studio, enabling developers to create agents that can work with robots to complete activities like financial reporting or customer support. This shows the transformative potential of AI agents as they can use advanced monitoring to continuously optimize their performance, improving ROI analysis and allowing businesses to integrate automation strategies effectively.
Explore how Agentic AI can transform your business
Reach Out for a ConsultationEnd Note
Agentic AI is still in its development stage and is expected to be more advanced. Its current potential is to empower businesses to be more efficient, agile and rapid. Everything is going to happen very quickly, and the business case for its adoption will also grow.
The incorporation of Agentic Automation in businesses needs thoughtful design, as there is no proper system that fits all sizes. Specialized AI agents will be created for jobs, and choosing the right tool might be a potential challenge. The enterprises should understand this new technology's hype and reality, promise and dangers.