Agentic Workflows
BLOG
The rise of Artificial Intelligence has changed how businesses think and act. With continuous advancements, agentic workflows have emerged as a transformative milestone in the automation revolution. Generative AI has been around for years now but often lacked contextual awareness, leading to outputs that may not align with specific tasks or enterprise environments. Agentic AI resolves this by incorporating decision-making and contextual understanding, enabling more relevant and actionable responses, serving as a foundation for agentic workflows.
These workflows are innovative automation systems that empower businesses to achieve more by seamlessly integrating intelligence into everyday operations. Agentic workflows interact with large language models (LLMs) and other AI tools to handle complex tasks with remarkable precision. Unlike traditional methods, these workflows adapt, learn, and deliver outputs that are significantly more accurate and efficient.
In this blog, we will decode the concept of agentic workflows, explore their core pillars, and learn how businesses can reap their full potential.
What Are Agentic Workflows?
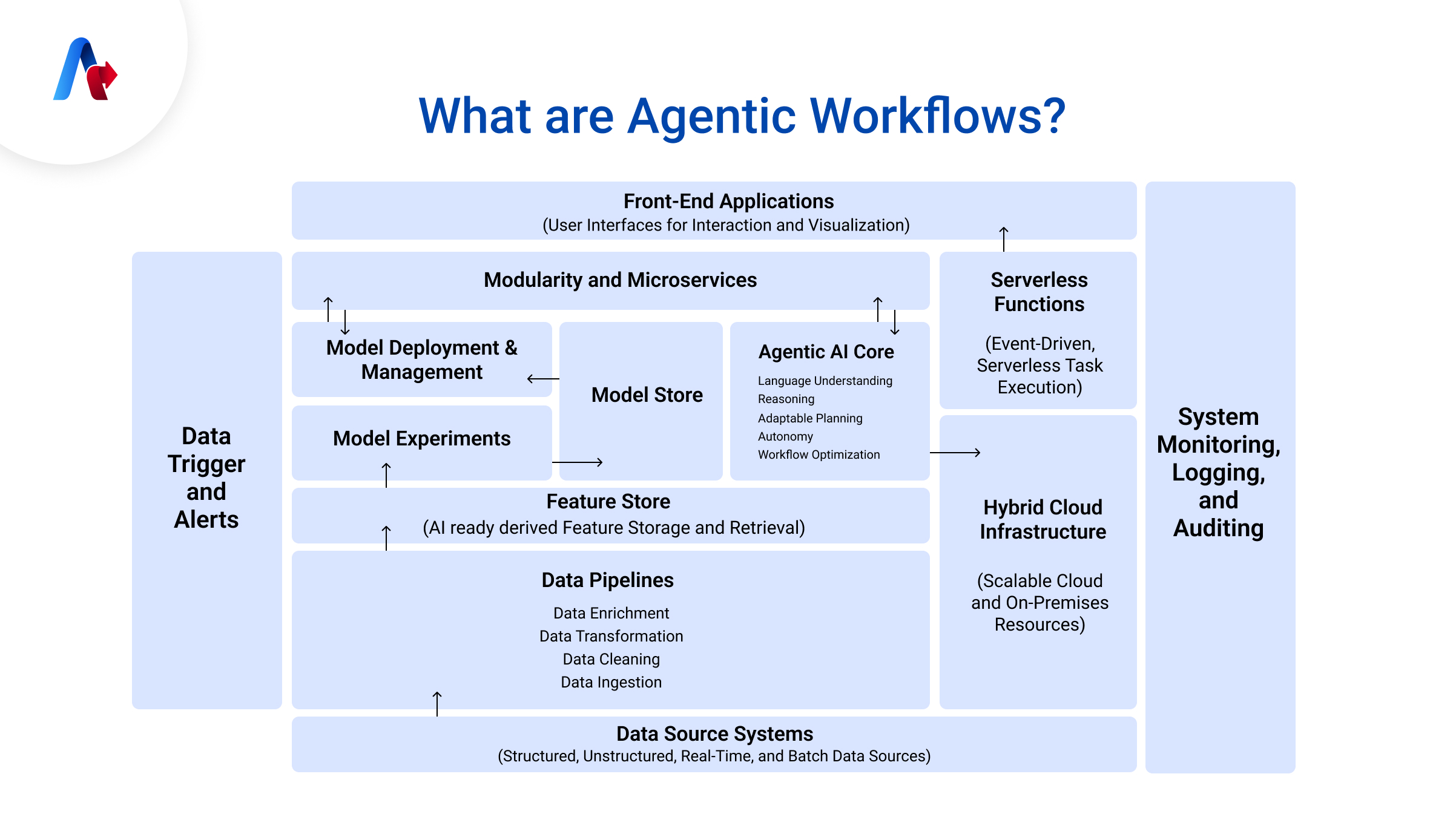
Agentic Workflows are automation systems that use AI agents to make informed decisions and execute tasks autonomously. These systems don’t rely on rule-based operations and act as virtual co-workers, adapting to dynamic environments, interacting, and freeing up humans to focus on tasks that bring the most value to the business.
Agentic workflows are also referred to as agentic processes or AI-agent-enabled processes, with the ability to reason, solve, choose the course of action, and execute. Unlike traditional AI applications that are utilized to process data and guide decision-making, these agentic workflows are designed to take action in real-time based on their analyses, making decisions and adapting processes to changing circumstances.
This type of workflow is common in systems where agentic AI needs to interact dynamically with its surroundings such as in robotics, game AI, smart manufacturing, and autonomous driving. Other use cases include personalized virtual assistants, adaptive cybersecurity systems, intelligent traffic management, automated financial trading, AI-driven healthcare diagnostics, and predictive maintenance in industrial operations.
Ready to experience the power of AI-driven agentic workflows?
Schedule a consultationAgentic Workflows vs. Traditional Workflows
Basis of Differentiation | Agentic Workflows | Traditional Workflows |
---|---|---|
Intelligence | Incorporates AI agents with contextual understanding and decision-making capabilities. | Operates on predefined, rule-based logic with no contextual adaptability. |
Adaptability | Adapts dynamically to changes in data, tasks, or environments. | Rigid and static; requires manual intervention for changes or updates. |
Automation Level | Combines intelligent automation tools like RPA and NLP to handle complex, unstructured tasks. | Limited to repetitive, structured, and rule-based tasks. |
Decision-Making | AI agents analyze data and make decisions autonomously, reducing reliance on humans. | Relies on humans for decision-making and problem-solving. |
Integration | Seamlessly integrates with multiple systems, APIs, and tools to ensure cohesive workflows. | Limited integration capabilities; often works in silos without smooth cross-system communication. |
User Interaction | Leverages NLP and conversational AI for seamless, natural user interactions. | Requires predefined input formats and lacks conversational capabilities. |
Scalability | Easily scalable to handle large volumes of data and complex processes. | Scaling requires significant reconfiguration and manual effort. |
Learning Capability | AI agents can learn from historical data and improve over time. | No learning capability; outcomes remain static unless explicitly reprogrammed. |
Error Handling | Identifies and resolves errors autonomously with minimal human intervention. | Errors often require manual intervention to diagnose and resolve. |
Examples of Use Cases | Customer service chatbots, predictive analytics, automated financial reporting, and decision support. | Invoice processing, basic data entry, and static approval workflows. |
Key Components of Agentic Workflows
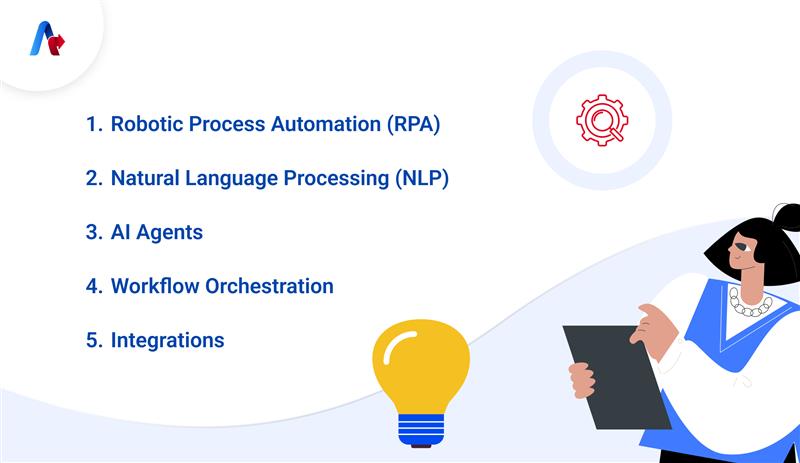
Agentic workflows are built on a foundation of intelligent automation, enabling secure and governed AI-driven processes. Here are the key components:
- Robotic Process Automation (RPA): RPA tools create software robots that mimic human actions such as data entry, transaction processing, and report generation. In agentic workflows, RPA enables AI agents to accurately execute rule-based tasks across applications. For example, RPA can automatically input invoice data extracted by an AI agent into an accounting system.
- Natural Language Processing (NLP): NLP allows AI agents to understand and respond to human language meaningfully and contextually. This eliminates the need for specialized training, making interactions with AI agents seamless. NLP is crucial for tasks like customer support, content generation, and sentiment analysis, enabling the creation of chatbots and conversational agents that engage with users in real time.
- AI Agents: AI agents are intelligent entities that perform complex tasks, make decisions, and learn from their interactions. Built on semantic engines or LLMs, AI agents can reason, plan, and interact conversationally. They use function calling to connect to systems, run automations, search for information, and complete goals, supported by a robust automation layer.
- Workflow Orchestration: Workflow orchestration coordinates tasks and components within agentic workflows. It defines action sequences, manages dependencies, and ensures tasks are executed efficiently. Orchestration tools provide visual interfaces for designing and monitoring workflows, integrating technologies and systems to manage complex processes seamlessly.
- Integrations: Integrations and APIs connect systems, applications, and technologies, enabling smooth communication and task execution. Agentic workflows rely on integrations to connect AI agents with tools like RPA and CRM systems, automating updates and ensuring cohesive processes, such as updating customer information for sales and support teams.
Practical Use Cases for Agentic Workflows
Agentic workflows offer immense value across various business functions. Here are some key practical applications or agentic workflows examples:
- Human Resources Management Agentic workflows can streamline HR tasks like recruitment, onboarding, and performance management. AI agents can automate resume screening to identify qualified candidates and schedule interviews, accelerating the talent pipeline and reducing biases in candidate selection. During onboarding, AI agents act as interactive guides to help new employee's complete forms, enroll in benefits, and navigate training modules.
- Project Management Traditional project management involves manual updates and communications, which can lead to delays and misalignments. Agentic workflows automate tasks like tracking project progress, providing real-time updates on milestones, sending alerts for potential bottlenecks, and reassigning tasks to balance workloads. For example, in software development, an AI agent can monitor task progress and allocate resources to meet deadlines efficiently.
- Customer Support AI agents in customer support enhance service quality by automating routine inquiries, providing personalized recommendations, and assisting service agents. NLP-powered agents handle FAQs, order tracking, and escalate complex issues to human agents when necessary. This hybrid approach improves response times and allows human agents to focus on resolving sensitive or unique problems.
- Finance Operations Finance departments can leverage agentic workflows to process large datasets, identify trends, and assess risks. AI agents analyze historical data and market conditions to make informed financial decisions, such as optimizing supplier discounts or ensuring SOX compliance. Additionally, AI agents can automate tax optimization processes, reducing liabilities and increasing savings.
Types of AI Agents
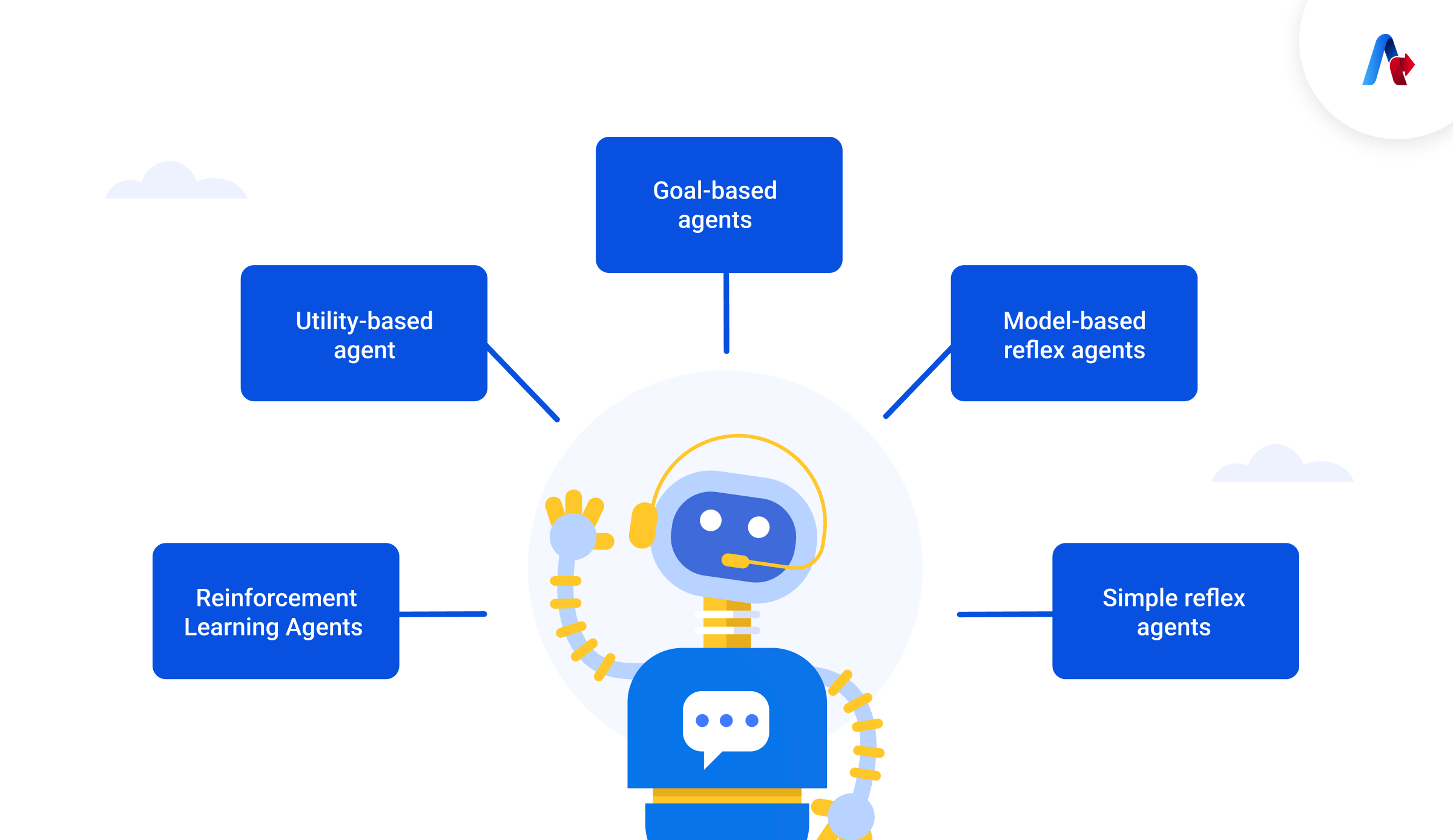
1. Reinforcement Learning Agents
Reinforcement learning (RL) agents operate by learning through trial and error, guided by rewards or penalties for their actions. Over time, they identify actions that maximize rewards, improving performance with each iteration. A notable example is AlphaGo, which mastered the game of Go by playing against itself and refining its strategies with every match.
2. Utility-based agent
These agents make decisions based on what gives them the best outcome. They calculate different choices and pick the one with the most benefits, like choosing the fastest and safest route on Google Maps. More examples include stock trading algorithms in which AI analyzes market conditions and selects trades with the highest expected profit while minimizing risk.
3. Goal-based agents
These agents work toward a specific goal. They take actions that bring them closer to achieving their goal, keeping the possible consequences for each action. For example, GPS navigation system that finds the best way to reach your destination, Medical AI analyzes symptoms and test results to find the best treatment plan for a patient, aiming for a correct and timely diagnosis.
4. Model-based reflex agents
These agents remember past situations in the form of past and current data and use that knowledge to make better decisions. They don’t just react but also consider what they learned before, like a self-driving car that remembers road conditions.
5. Simple reflex agents
These agents react instantly based on set rules without thinking about the past. They work like a traffic light that changes based on sensor input, without considering past traffic patterns. More examples include a smart thermostat that detects room temperature and automatically turns the heater on or off without remembering previous temperatures.
Benefits of AI Agentic Workflows
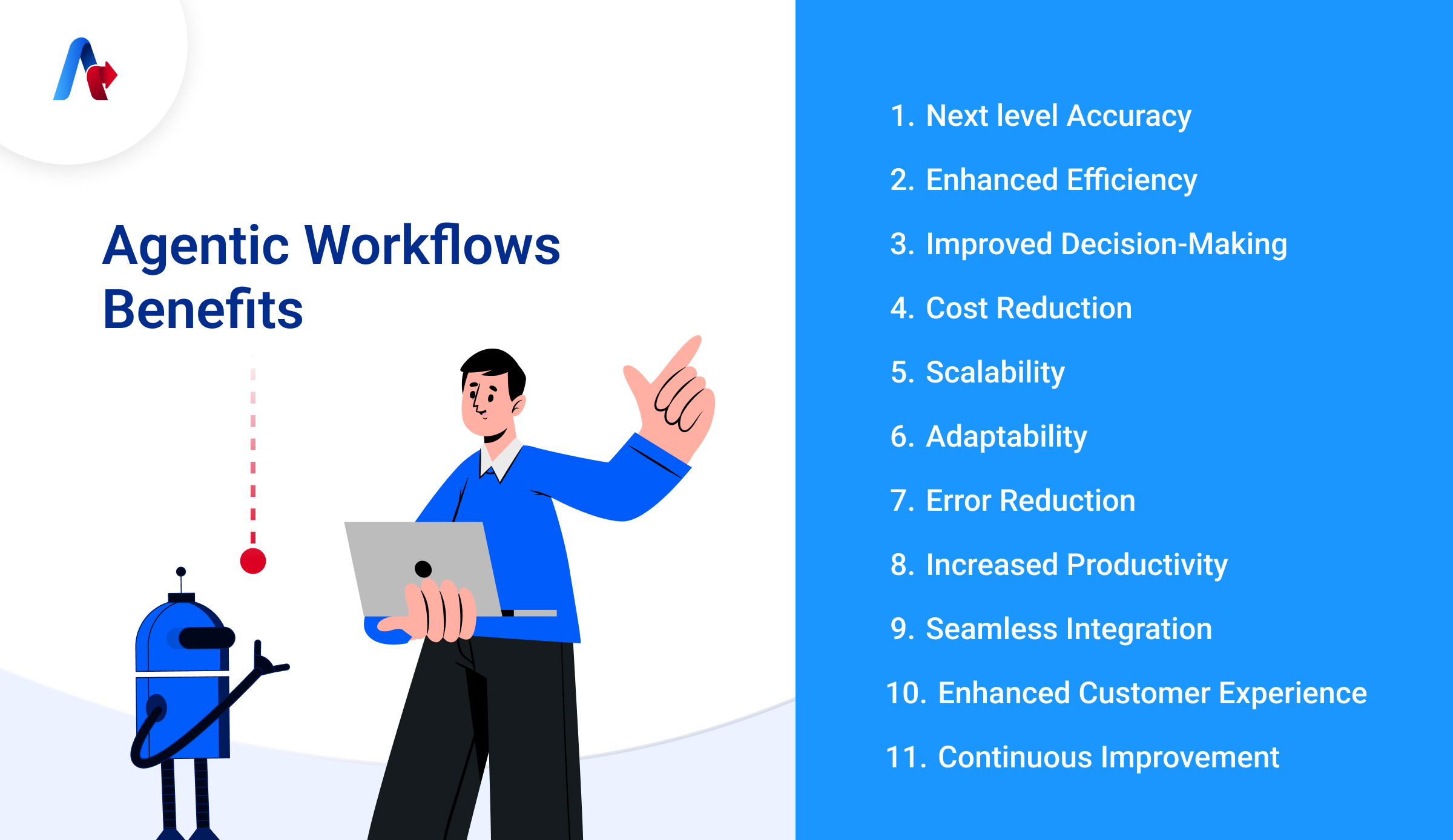
Agentic workflows extend efficiency gains by delivering extensive, scalable, and autonomous process support. Let’s take a look at what it looks like from an enterprise standpoint:
1. Next level Accuracy
Agentic workflows ensure that discrepancies are identified and addressed right away, either by an AI agent within the workflow itself or by triggering human-in-the-loop processes to surface exceptions for human review and input.
2. Enhanced Efficiency
Automates repetitive and time-consuming tasks, allowing businesses to streamline operations and achieve faster results.
3. Improved Decision-Making
AI agents analyze real-time data, providing actionable insights and enabling more informed and accurate decisions.
4. Cost Reduction
Minimizes operational costs by reducing reliance on manual labor and improving resource allocation.
5. Scalability
Handles increasing workloads seamlessly without compromising performance or accuracy, making it ideal for growing businesses.
6. Adaptability
Dynamically adjusts to changing environments, processes, or data inputs, ensuring workflows remain relevant and effective.
7. Error Reduction
Decreases human errors by automating complex processes and improving data accuracy.
8. Increased Productivity
Frees up human resources to focus on strategic and creative tasks, enhancing overall productivity.
9. Seamless Integration
Connects multiple systems, tools, and platforms for end-to-end process optimization and smooth communication.
10. Enhanced Customer Experience
AI agents deliver personalized and timely interactions, improving customer satisfaction and loyalty.
11. Continuous Improvement
Leverages machine learning to refine processes over time, ensuring workflows become smarter and more efficient.
Let AI agents optimize processes while you focus on success
Talk to AI expertsExperience the Impact of Agentic Workflows Firsthand
Deploying agentic workflows helps enterprises automate complicated and effort-intensive processes. As automation technologies evolve, these agentic workflows' use cases will increase and address real-world enterprise challenges across various industries. Accelirate is a trusted platinum UiPath partner and a leading expert in agentic automation. Our dedicated team can help you leverage the full capabilities of agentic workflows.