Agentic AI Architecture
BLOG
10 min read
Understanding Agentic AI Architecture: Key Components and Their Impact on AI Efficiency
With the use of agentic AI architecture, Artificial Intelligence systems can operate independently, make choices, establish objectives, and carry out tasks without continual human supervision. Agentic AI actively interacts with its surroundings to learn from data, and adjust in real-time, compared to traditional AI.
Industries like healthcare, finance, manufacturing, and retail leverage the power of Agentic Automation to drive automation and efficiency.
Check this blog and explore how agent architecture works, its components, types, and how industries are making the best use of this technology.
What is Agentic AI Architecture?
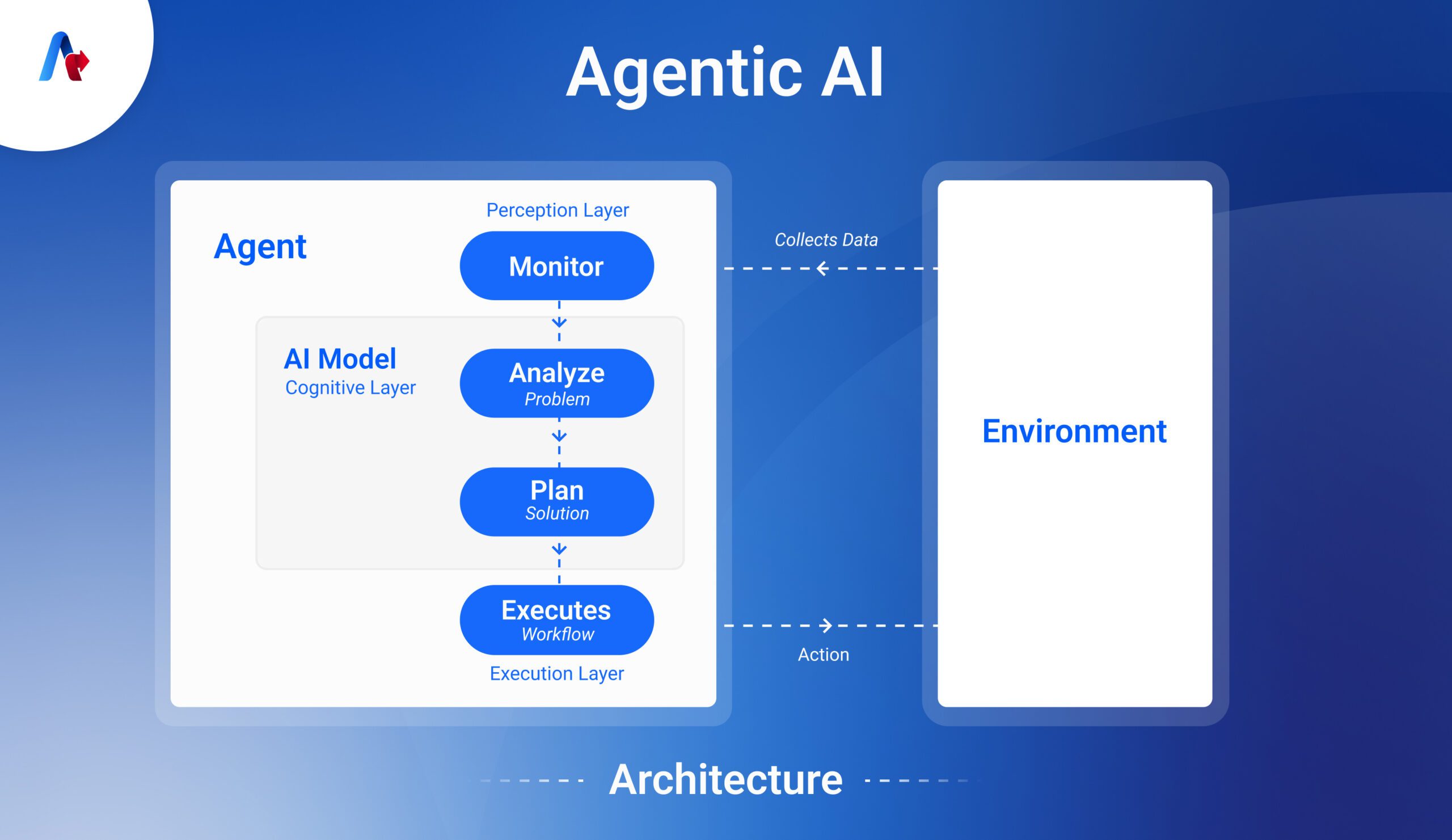
The term "agentic AI architecture" describes artificial intelligence systems built to function as independent agents that can decide for themselves, define objectives, and carry out activities on their own. Agentic AI actively engages with its surroundings, collects data, and adjusts in response to real-time feedback, in contrast to classic AI models that passively react to inputs.
These systems manage complicated tasks without constant human supervision by utilizing memory, adaptive techniques, and multi-step reasoning. As per a report by Gartner, by 2029, 80% of common customer service issues will be resolved by Agentic AI autonomously.
Agentic AI is able to analyze events, predict difficulties, and optimize results in dynamic scenarios by combining sophisticated machine learning models, natural language processing, and reinforcement learning.
How Agentic AI Architecture Works?
By fusing several AI capabilities, Agentic AI architecture produces autonomous systems that are able to sense their surroundings, make judgments, and take action to accomplish predetermined objectives. Perception, reasoning, planning, action, and adaptation are all fundamental components of the structured workflow that underpins agentic AI.
It starts with collecting data using computer vision, natural language processing (NLP), or machine learning models from a variety of sources, like user inputs, sensors, and databases. After processing this information, the AI uses reasoning skills to create a plan to complete a task.
Large language models (LLMs) and reinforcement learning are examples of advanced models that assist the system in assessing several strategies and selecting the best one.
Regardless of the industry that your company belongs to, integrating Agentic AI will boost the performance and results.
The image below shows why is it important for businesses to consider early adoption of Agentic AI.
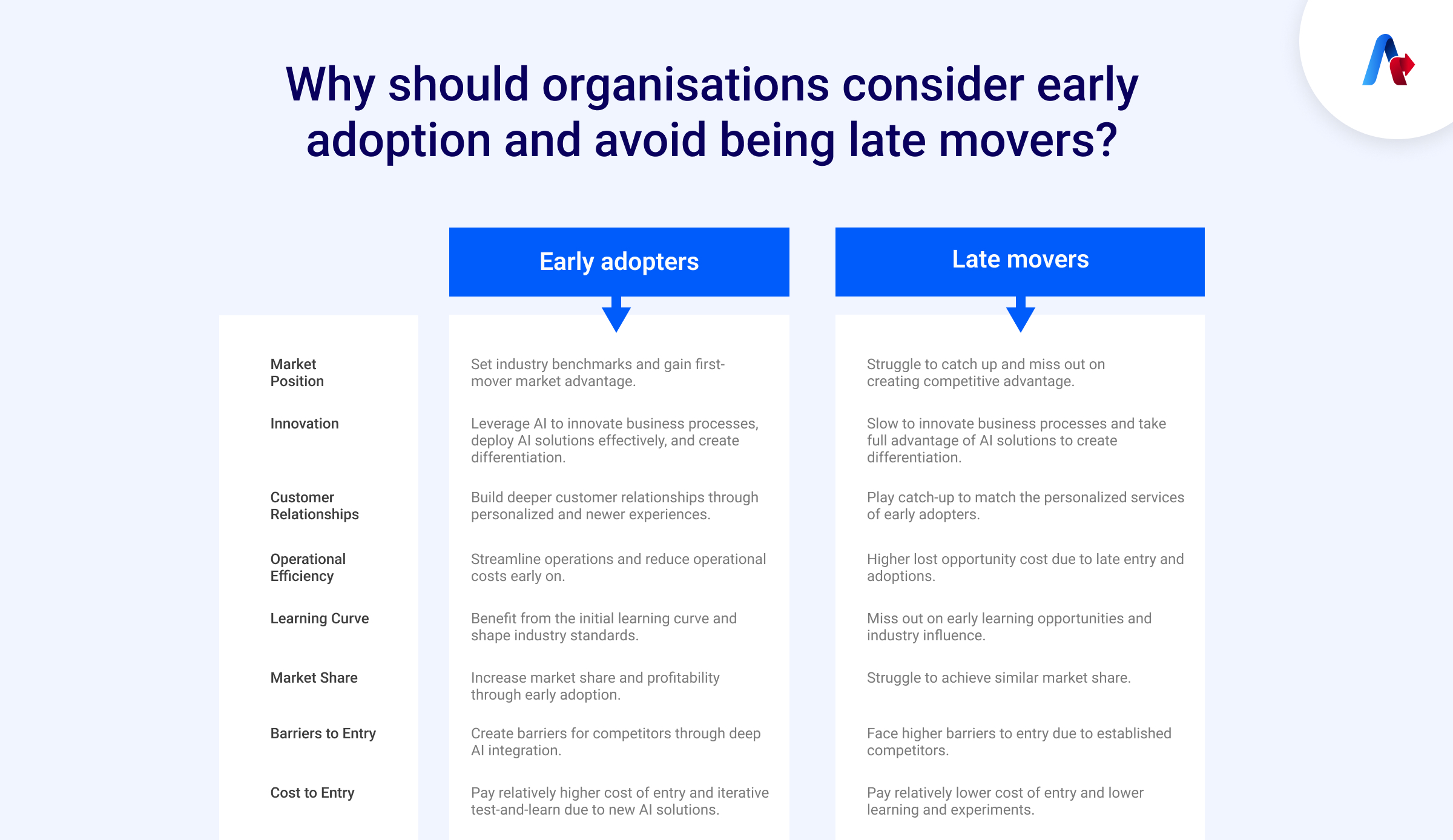
Key Building Blocks of Agentic AI Architectures (Components)
The basis of agentic AI architecture is a collection of fundamental elements that provide AI systems the ability to function independently, make wise judgments, and adjust to shifting conditions. Together, these components enable the AI to see, think, act, and gain knowledge from its experiences.
Perception and Data Processing
- This section enables the Artificial Intelligence to integrate and examine data from text, images, sensors, databases, and user participation.
- For effective understanding and analysis, it consists of computer vision, speech recognition, and natural language processing (NLP).
- Example: A chatbot employs text analysis to evaluate the user’s question prior to picking an appropriate answer.
Memory and Learning System
- It helps the AI perform better over time by storing key information, learned patterns, and previous interactions.
- Retains relevant data for improved decision-making by using both short-term and long-term memory strategies.
- Example: A financial AI assistant can recall a user's investment preferences and make personalized stock recommendations based on previous selections.
Reasoning and Decision-Making Engine
- It evaluates incoming information, applies reasoning, and chooses the optimal path of action.
- Evaluate several possibilities and select the best one using AI models such as rule-based systems, reinforcement learning, and large language models (LLMs).
- Example: An autonomous trading bot uses real-time data to execute buy/sell orders and analyze market patterns.
Action Execution and Automation
- Converts choices into practical actions, like messaging, transaction processing, workflow automation, and physical system control.
- Ensures seamless execution by integrating with databases, external systems, and APIs.
- Example: Based on user requests, an AI-powered virtual assistant plans meetings, arranges travel, and automatically responds to emails.
Feedback Loop and Adaptation
- Keeps an eye on the outcomes of its actions, requests input, and gradually refines its tactics.
- It uses strategies such as real-time data analysis and self-learning models to improve decision-making and maximize subsequent actions.
- Example: A customer service AI gradually increases the accuracy of its responses by refining them in response to user satisfaction scores.
Discover how our customized agentic AI solutions can drive ROI and operational efficiency.
Talk to Our Automation ExpertsComparing the Different Types of Agentic AI Architectures
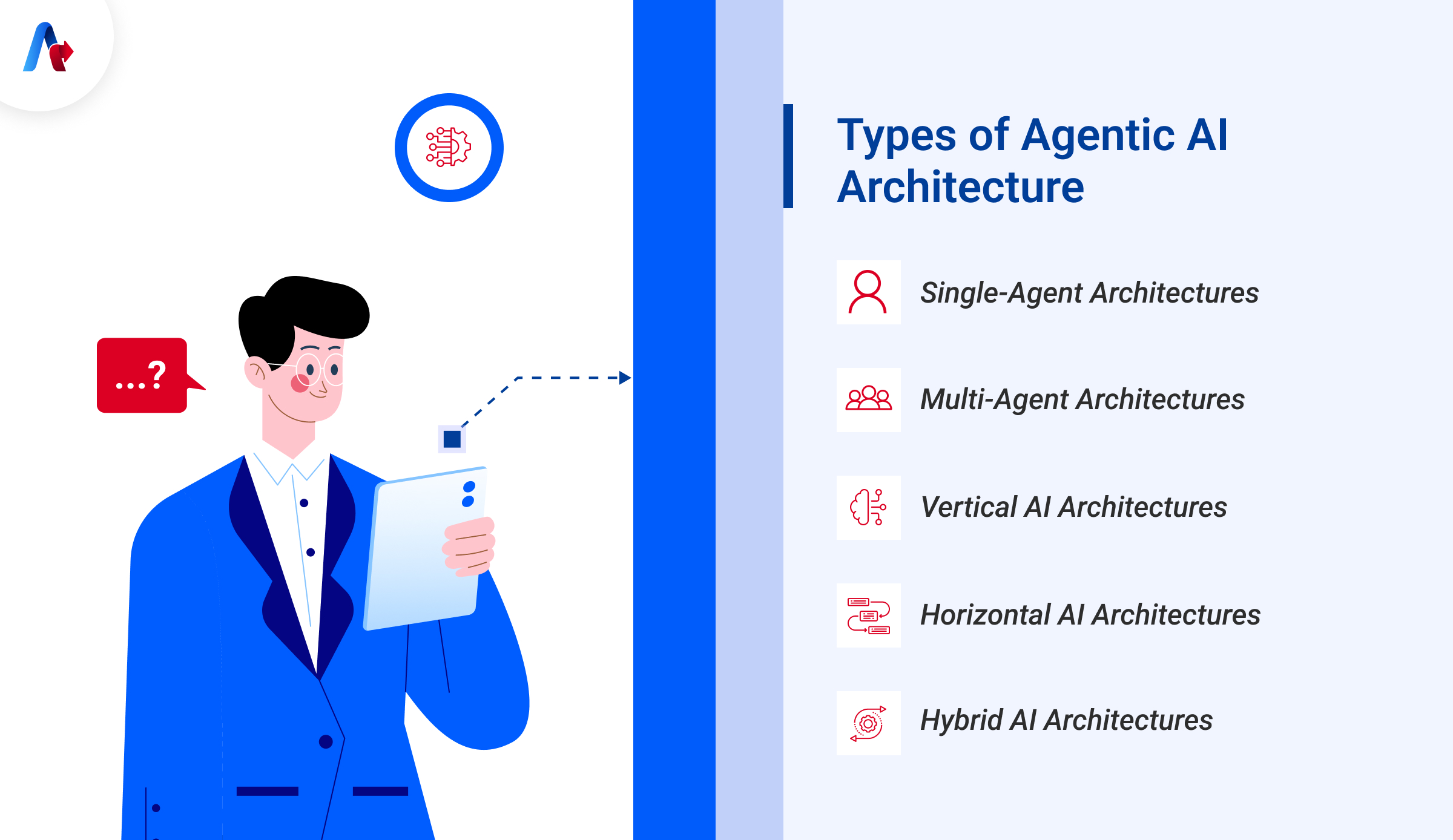
Different agentic AI architectures have different collaboration models, decision-making procedures, and structural differences.
01. Single-Agent Architectures
In a single-agent architecture, an autonomous AI unit functions independently in its surroundings. Without consulting other agents, this AI agent interprets inputs, decides what to do, and acts. It works best for independent projects when detailed teamwork is not required.
Key features
- Functions autonomously without outside assistance.
- Centralized execution and decision-making.
- Direct command over the course and results of the task.
02. Multi-Agent Architectures
In multi-agent architectures, several AI agents collaborate, each with a unique job or task of expertise. Compared to a single-agent system, these agents work together and interact to solve complicated issues more quickly. This architecture is perfect for large-scale operations and dynamic contexts because it improves flexibility, scalability, and adaptation.
Key features
- Several agents cooperated in a coordinated manner.
- Decision-making that is distributed or decentralized.
- The capacity to exchange knowledge and adjust to shifting circumstances.
03. Vertical AI Architectures
A leader agent supervises and controls other subordinate agents in a hierarchical vertical AI system. In addition to assigning tasks and making high-level decisions, the leader ensures that the agents function effectively within a defined framework. For workflows that need defined responsibilities and accountability, this architecture is helpful.
Key features
- A hierarchical structure with clearly defined duties.
- Central authority from a leader agent.
- The way tasks are executed is top-down and structured.
04. Horizontal AI Architectures
All agents function as equals and freely cooperate in a horizontal AI architecture, which is decentralized and lacks a hierarchical framework. Every agent plays an equal part in decision-making, helping to solve problems and carry out tasks. Tasks requiring creativity, invention, and adaptability are a good fit for this model.
Key features
- Peer-based, decentralized cooperation.
- Agents share decision-making.
- Parallel processing allows tasks to be completed simultaneously.
05. Hybrid AI Architectures
Both decentralized cooperation and structured leadership are made possible by a hybrid AI architecture, which blends aspects of vertical and horizontal systems. As leadership responsibilities can change according to the demands of the task, this model is very flexible and able to manage both dynamic and organized workflows.
Key features
- Situation-specific leadership that is adaptable.
- A mixture of decentralized and centralized approaches.
- Achieve equilibrium between innovation and effectiveness.
Applications of Agentic AI Architecture Across Various Industries
Agent architecture is now a part of every industry. From helping businesses scale to ensuring better decision-making, these agents have proved their worth. Here is how Agentic AI Architecture is helping various industries:
Industry | Primary Applications | Key Benefits |
---|---|---|
Manufacturing | Autonomous robots, Quality control | Enhanced efficiency, Reduced errors |
Healthcare | Diagnostic systems, Patient monitoring | Improved accuracy, 24/7 monitoring |
Transportation | Autonomous vehicles, Traffic management | Reduced accidents, Optimized flow |
Finance | Algorithmic trading, Risk assessment | Fast execution, Better risk management |
Retail and eCommerce | Personalized recommendations, Inventory management | Increased sales, Reduced stock issues |
Real Estate | Property valuation, Smart home automation | Accurate pricing, Enhanced security |
Healthcare
By facilitating more precise diagnostics, optimizing administrative processes, and customizing patient care, agentic AI systems are transforming the healthcare industry. Multi-agent systems work together to evaluate medical imaging, track patients' vitals in real-time, and help physicians make decisions.
Finance
Agentic AI is utilized in finance for automated trading, risk evaluation, and fraud detection. AI agents collaborate to keep an eye on transactions, identify questionable activity, and improve investment plans. Real-time analysis of large datasets by these systems guarantees prompt and precise decision-making in unpredictable marketplaces.
Manufacturing and Supply Chain
By facilitating predictive maintenance, streamlining processes, and real-time supply chain management, agentic AI increases manufacturing efficiency. As per a report by McKinsey and Company, AI in the manufacturing sector helps reduce production costs by 15%-20%.
Transportation and Logistics
By controlling traffic systems, streamlining delivery routes, and facilitating self-driving technology, agentic AI improves transportation efficiency. In logistics firms, AI agents work together to guarantee on-time delivery, cut fuel usage, and simplify fleet management.
Real Estate
Through virtual property tours, AI-powered agentic systems assist real estate agents in evaluating property prices, examining market trends, and improving client interactions. AI-powered platforms give investors, buyers, and sellers data-driven insights to help them make better decisions.
Retail and eCommerce
Businesses employ Agentic AI to enhance customer service with AI chatbots, assist in inventory management, and automate product offer suggestions. The use of AI technologies makes the shopper’s experience issue-free by analyzing user activity, optimizing the supply chain, and anticipating needs.
Take Amazon as a case study. The company applies LLM agent architecture to offer more accurate and custom-tailored recommendations to its clients.
What's Your Use Case?
We have a solution
for you
Get Expert Consultation
How Agentic Architecture Is A Game-Changer?
It is no secret that we are shifting towards more complex systems and structures, especially when we talk about the economic space. Business activity becomes more decentralized, and Agentic AI is one of the key drivers to achieve such a transformation. When an AI system operates on its own and is able to make choices and optimize actions with minimal human involvement, we define that as agentic design.
From healthcare to banking, retail, and logistics – companies across industries are embracing the shift toward automation and leveraging emerging technologies to drive efficiency and innovation. As the global economy moves rapidly toward hyper-automation, adopting Agentic AI is no longer optional — it's essential for staying competitive.
Accelirate, an enterprise Agentic platform, is here to help you design, develop, and embed agentic architecture tailored to your business needs. Let us guide you on the path to intelligent automation. Contact us today to kickstart your agentic AI journey and future-proof your operations.
FAQs
Healthcare, banking, and logistics need AI systems that work on their own, which is pushing the quick uptake of AI agents. The global agentic AI architecture is projected to reach USD 49.6 billion by 2034.
An agentic design pattern is a behavioral interface that allows an AI system to autonomously observe, reason, formulate, and execute plans independently. In other words, it empowers AI bots to collaborate, reconfigure, and make complex decisions without constant supervision.
Healthcare, robotics, banking, autonomous vehicles, and virtual assistants are some of the domains that have adopted agentic AI. In crucial industries, it enables AI-powered decision-making, automation of processes, supply chain management, and personalization.