AI Agent Orchestration
BLOG
12 min read
AI Agent Orchestration: Managing Multi-Agent Systems for Business Efficiency
How do enterprises keep up with the growing complexity of automation and decision-making in this decade ruled by Artificial Intelligence? The answer is AI agents and their smooth orchestration with multi-agent systems.
What are AI agents? These are intelligent software sets that facilitate specific activities, from Customer Interactions to advanced Data Analytics. Algorithms and machine learning models drive them to make choices, emphasize adaptive learning and contribute actions without constant human support. Their ongoing prominence in automation is beyond thoughts; as Gartner states 75% of organizations are going to integrate AI-given solutions within their workflows by 2026, capitalizing on the transformative power of AI systems.
Businesses have multiple processes and agendas, demanding more than one agent to manage different operations. This calls for Multi-Agent Systems (MAS), the net worth of different AI agents working as a team to reduce challenges. From customer service orchestration to logistics optimization, MAS allows more scalability, effectiveness and precision in managing complex business environments.
Apart from its advantages, managing MAS is challenging. Without the prevalence of an AI agent orchestration framework, businesses won't be able to harness the transformative potential of multiple agents at work. It becomes more complicated as individual agents operate on different data sets, resulting in disparate predictions or outcomes. This involves the demand for intelligent orchestration, which enables agents to share data contextually and facilitate smooth task transitions.
The significance of coordination and scalability in multiple agent systems cannot be ruled out. A well-defined orchestrated system will optimize workflows, enhancing decision-making by unifying agents under a single operational framework.
In this blog, we will examine different approaches to AI agent orchestration, its advantages and real-world use cases, which are transforming different industries today.
What is AI Agent Orchestration?
AI Agent Orchestration refers to coordinating multiple AI agents within a unified system to ensure the performance of each AI agent within their defined areas and accomplish shared objectives. It implements intelligent orchestration to monitor agents powered by large language models like GPT-4, Claude or Gemini. These agents can manage business operations like customer support, inventory management, and troubleshooting.
Multiple agent orchestration allows effective communication between agents, enabling sharing relevant data across activities without interruption. Let's consider a customer service scenario where an AI agent manages billing queries, and in case of any issue, technical support is required. However, multi-agent orchestration automatically transitions the activity to a particular troubleshooting agent. This is what the integration looks like: securing adaption, efficiency and contextual accuracy in real-life scenarios.
In simple terms, agent orchestration frameworks act as a foundational component for businesses looking to scale automation while managing quality and customized service.
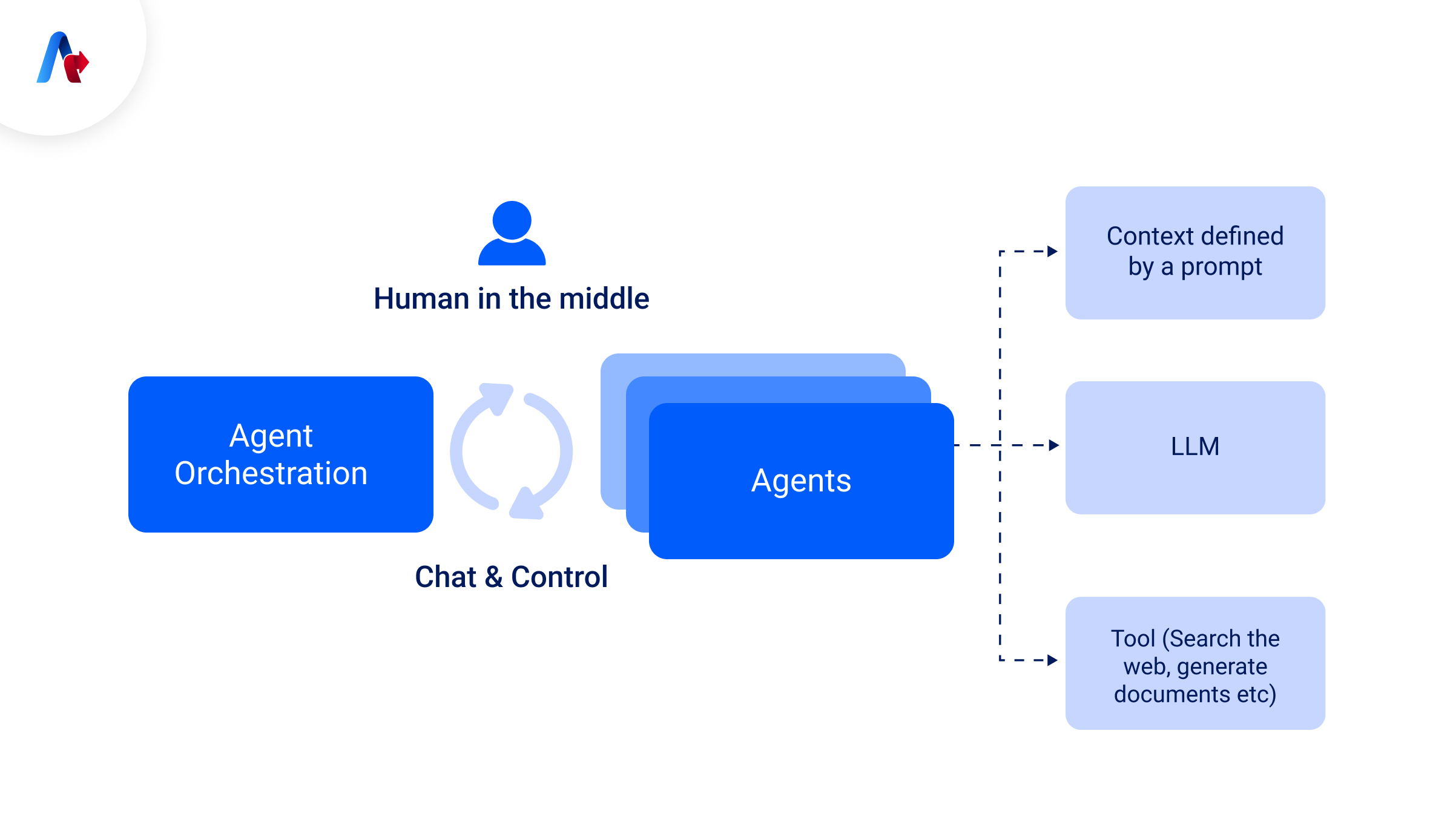
Benefits of AI Agent Orchestration
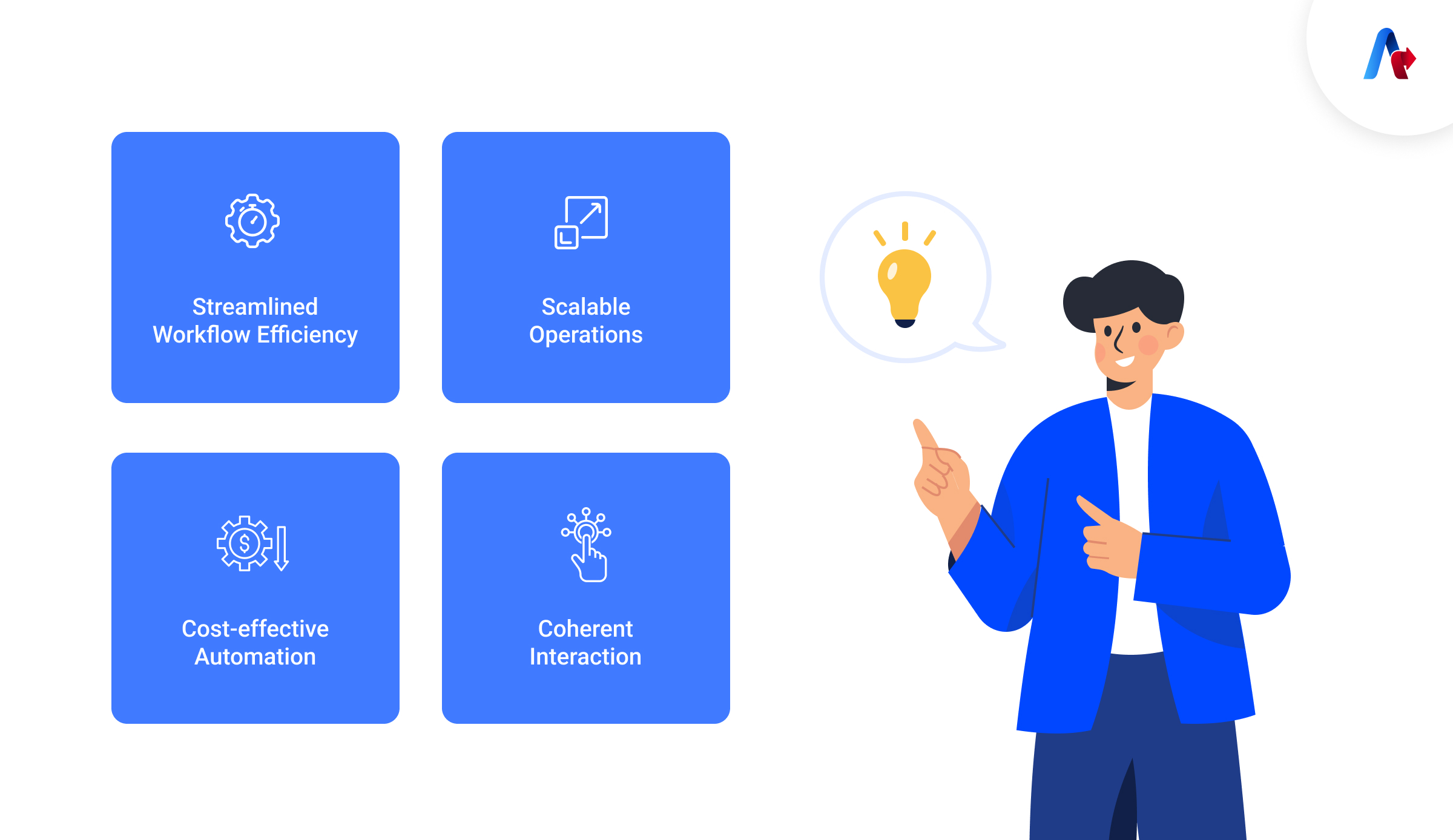
AI agent orchestration offers the latest edition to your business efficiency, unlocking distinct strategic advantages with the synchronized utilization of multiple AI agents.
Let's consider the benefits:
- Streamlined Workflow Efficiency: AI orchestration offers task specialization by emphasizing agents based on their functions, such as billing, product recommendations, and troubleshooting, and enhancing the clarity of responses and rapid task completion.
- Scalable Operations: These orchestration frameworks allow easy expansion, helping businesses to incorporate additional AI agents as demand grows in domains like customer service or logistics, causing no disruptions in existing operations. Forrester has highlighted that, 56% of business has experienced an increase in scalability while implementing AI orchestration frameworks.
- Coherent Interaction: With multi-agent orchestration, agents can share relevant information across domains, contributing to contextual consistency in customer interactions and a smooth user experience. PwC reports the Importance of consistent communication, as 75% of customers expect it across different touch points, which are empowered by orchestration frameworks as it shares information between agents.
- Cost-effective Automation: Intelligent Orchestration enables businesses to offer more personalized services by ensuring the understanding of agents from past customer interactions, improving customer engagement and retention.
Key Components of a Multi-Agent System
Let's investigate the breakdown of the key components of MAS:
Component | Description |
---|---|
Agents | Refers to an autonomous entity able to make decisions and perform activities depending upon structured goals and objectives. Agents are provided with adequate knowledge and decision-making capabilities. |
Communication protocols | Refers to the approaches, through which agents share information. The common protocols include foundation for Intelligent Physical Agents- Agent Communication Language (FIPA-ACL) or other customised protocols. |
Environment | Refers to the common space, where agents operate and interact. It can be physical or virtual, providing content for intelligent data agents to make decisions like real time updates on inventory. |
Coordination mechanisms | Involves the techniques to enable agents to work together without any conflict. The general examples comprise negotiation protocols, consensus foundation algorithms that maximizes collective performance. |
Agent interaction models | Structures the format on how agents are collaborating or competing with one another. For instance, utilization of market-based approaches to minimise conflicts and streamline resources. |
Scalability framework | Refers to the systems that facilitate agents with scaling possibilities, with the increase of agents in a system. It can involve decentralized decision making or distributed computing models. |
Data integration layers | Refers to the approaches that process data from different sources within a common format helping agents to make informed decisions depending on incorporated inputs particularly in complex environments like retail or logistics. |
Learning algorithms | Involves advanced learning with time, to enhance decision making capabilities of an agent based on experience or feedback like neural networks or reinforcement learning. |
Discover how AI agent orchestration can streamline your operations and drive scalability.
Contact Us NowSteps to Implement AI Agent Orchestration
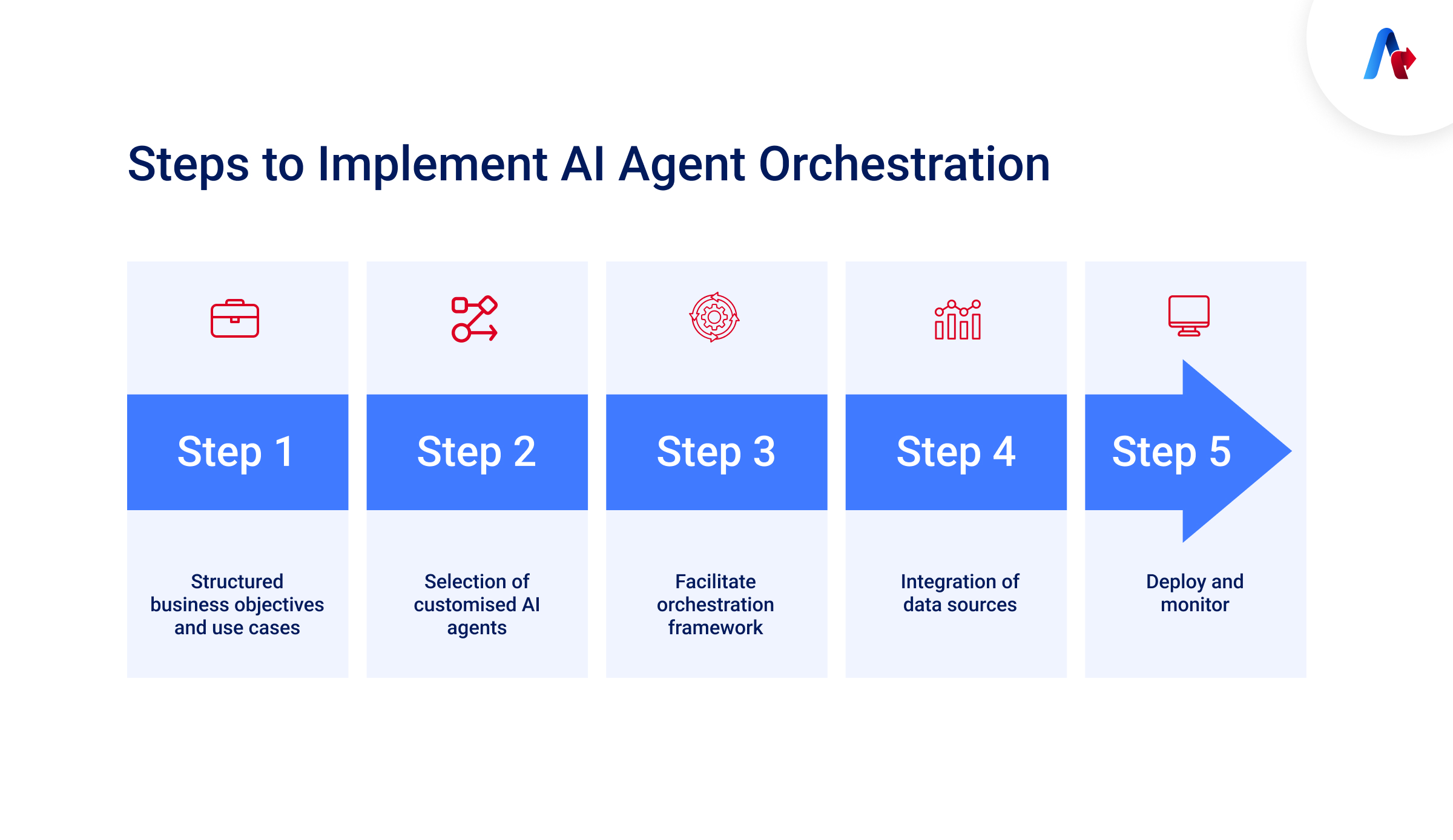
Implementing AI agent orchestration requires a structured approach to enhance performance and equip multiple agents with seamless integration in a business workflow.
1. Structured business objectives and use cases
Identify business challenges and processes that can benefit from agent orchestration, including customer service and logistics management.
2. Selection of customised AI agents
Agents need to be chosen based on their tasks, such as troubleshooting, inventory management or billing. These agents should utilize large language models like Anthropic Claude or GPT-4 for advanced functionality.
3. Facilitate orchestration framework
Involve an AI agent orchestration framework, including Data Exchange, Communication protocols (FIPA-ACL) and context dependency across agents.
4. Integration of data sources
Process contextual data from different sources and ensure that agents can access updated and synchronized information to make informed choices.
5. Deploy and monitor
Implementing an orchestration system while continuously monitoring its performance using AI analytics and real-time monitoring tools to track coordination and scalability.
Example of an Orchestrated AI agent
To consider an example of orchestrated AI agents, let's examine a telecommunications environment in which the AI agent system is placed within the customer support industry.
- Billing agent: The customer initially interacts with a billing agent who facilitates account inquiries, payment concerns and subscription management. The agent implements large language models like GPT 4 to identify and resolve queries.
- Technical support agent: In case the customer requires assistance with service concerns like internet connectivity or other issues, the billing agent passes over the interaction to the technical support agent, who can pursue troubleshooting using device diagnostics and network management tools.
- Orchestrator: This is responsible for smooth transitions between agents, managing information with both agents and efficiently resolving concerns. It emphasizes maintaining the context and allowing an uninterrupted customer experience.
Use Cases of AI Agent Orchestration in Business
Customer Support:
AI agent orchestration is highly influential in customer support, as it allows for the streamlined management of different customer queries and the integration of specialized AI agents.
- Consider a retail organization that deploys an account management agent to address basic concerns while a fraud detection AI agent tracks complex issues like suspicious account handling.
- The Orchestrator helps smooth transitions between agents, providing context and enhancing the customer experience.
- Implementing such AI-enabled systems in customer support has been proven beneficial. Salesforce suggests that 92% of service teams implementing AI reports have reduced expenses, and 83% of sales teams with AI have documented revenue growth in 2023.
Supply Chain Management
AI agent orchestration is vital in supply chain management, as it optimizes inventory, logistics, and demand forecasting with intelligent coordination. These agents are provided with adequate information to manage activities like inventory management, shipment tracking and supply-demand matching, with orchestration capabilities to ensure that these agents work together in a unified system.
- AI agents track stock levels, updating the system to eliminate stockouts or overstock situations. They also communicate with demand forecasting agents to accommodate orders.
- AI agents also optimize routes, monitor delivery schedules and allow timely shipments by interacting with inventory systems to accommodate logistics based on real-time inventory levels.
- AI Agents analyze historical data and the latest market trends and use the information in production planning systems. They work continuously to predict levels and anticipate demand fluctuations.
Financial Services
Within financial automation services, AI agent orchestration streamlines operations like fraud detection, assessment and tailored recommendations through the integration of different specialized agents who work in unison.
- AI agents can process transaction patterns, detecting suspicious activities across accounts in real-time. Orchestration helps in seamless communication with other agents, speeding up decision-making and accuracy.
- AI agents monitor data from different sources to identify credit scores, loan risk factors and market trends. The orchestration of agents enables real-time risk reduction, allowing businesses to make informed decisions rapidly.
- Customer-facing agents examine individual preferences and goals to provide personalized financial plans and product recommendations. These agents can analyze customer data and portfolio performance, providing context-aware recommendations. According to McKinsey, AI-driven solutions in the financial services industry reduce operation expenses by up to 30% while enhancing fraud detection by 50% to 80%.
Healthcare
Within the healthcare sector, AI agent orchestration plays a critical role in managing patient data, scheduling and diagnosis. It enables seamless coordination across different functions for better operational efficiency and patient care.
- AI Agents can analyse and store patient data across EHRs, providing Healthcare providers with accurate and updated information.
- AI agents work in unison to automate appointment scheduling by analysing doctor availability, patient preferences and other factors, such as urgent medical conditions. Orchestrated agents enhance the patient experience by streamlining waiting times and reducing appointment conflicts.
- Diagnostic AI agents process medical images, patient symptoms and lab results. Orchestration helps multi-agent systems collaborate, maximizing diagnostic accuracy by cross-referencing information across departments or specialists.
Take the first step towards implementing AI agent orchestration for your business.
Schedule a consultation todayChallenges in Managing Multi-Agent Orchestration Systems
Managing multi-agent systems has specific challenges that must be mitigated with proper care and supervision. The primary importance is identifying the bottlenecks that cause enterprise inefficiencies:
- Complex coordination: Maintaining communication and synchronization with different agents is complicated, specifically when handling dynamic and other activities.
- Scalability: With the increased number of agents, managing them becomes complex as systems must handle additional workloads without any scope of degradation in performance.
- Data Privacy and Security: AI agents, particularly in the Healthcare or finance domain, can be challenging when it comes to secure and compliant data sharing. In 2023, 80% of Enterprises cited data security as a primary challenge to AI implementation.
- Integration: Incorporation of different agents with prevalent legacy systems is challenging as it requires significant effort and resources.
The Future of AI Agent Orchestration
Well, the future of AI agent orchestration in today's world is completely linked with the advancement of large language models and reinforcement learning. The increasing demand for scalable and adaptive systems powers up orchestration to support multi-agent coordination across different platforms, allowing teal-Time decision-making and continuous learning.
By integrating cloud-native technology and edge computing, Orchestration platforms will reduce latency and enhance performance. With AI-enabled monitoring and automated conflict resolution, the agent performance will be added to peak. Talking of challenges, the concerns over AI ethics and security frameworks will become more critical. Projections highlight a 30% increase in AI orchestration adoption by 2027, enabling this domain to reshape customer experience and operational efficiency.
Conclusion
The power of AI agent orchestration and its role in transforming business processes by increasing efficiency, scalability and customer satisfaction has yet to reach its full potential.
At Accelirate, we harness the power of AI and Automation to deliver intelligent orchestration, seamlessly integrating specialized AI agents to achieve optimal outcomes for our clients. With our expertise in Agentic Automation, we ensure that AI agents work collaboratively, switching responsibilities efficiently to drive innovation, streamline processes, and enhance decision-making. Let us guide you through the transformative journey of AI agents and their role in shaping the future of business operations.
FAQs
AI agent orchestration will significantly benefit customer support, Financial Services, health care, and supply chain management.
Traditional automation involves linear workflows, whereas an AI agent orchestration strategy incorporates specialized AI agents to enable dynamic decision-making and real-time cooperation across systems, resulting in greater scalability and adaptability.
UiPath has powerful orchestration tools, like UiPath Orchestrator, that allow Enterprises to manage and coordinate multiple AI agents across workflows.
The success of an AI agent orchestration strategy can be assessed through key performance indicators like response time, task completion rate, system efficiency and customer satisfaction.