Machine Learning and Automation Monitoring
Machine learning is an exciting concept. A machine ‘learns’ to perform certain tasks, ranging from sending automated emails to predicting issues and preventing them before they occur. This innovative technology has been driving automation monitoring towards a more proactive approach.
This article will discuss how machine learning can be used for automation monitoring also how it can benefit businesses in various industries.
What Is Automation Monitoring?
Just like Team managers oversee their teams to ensure a smooth running of things, automation monitoring oversees the automated systems responsible for performing repetitive tasks in a business setting.
This includes tasks like inventory management, order processing, and data entry. Automation monitoring aims to ensure that these systems work with maximum efficiency & zero error rate.
Why Is Automation Monitoring Important?
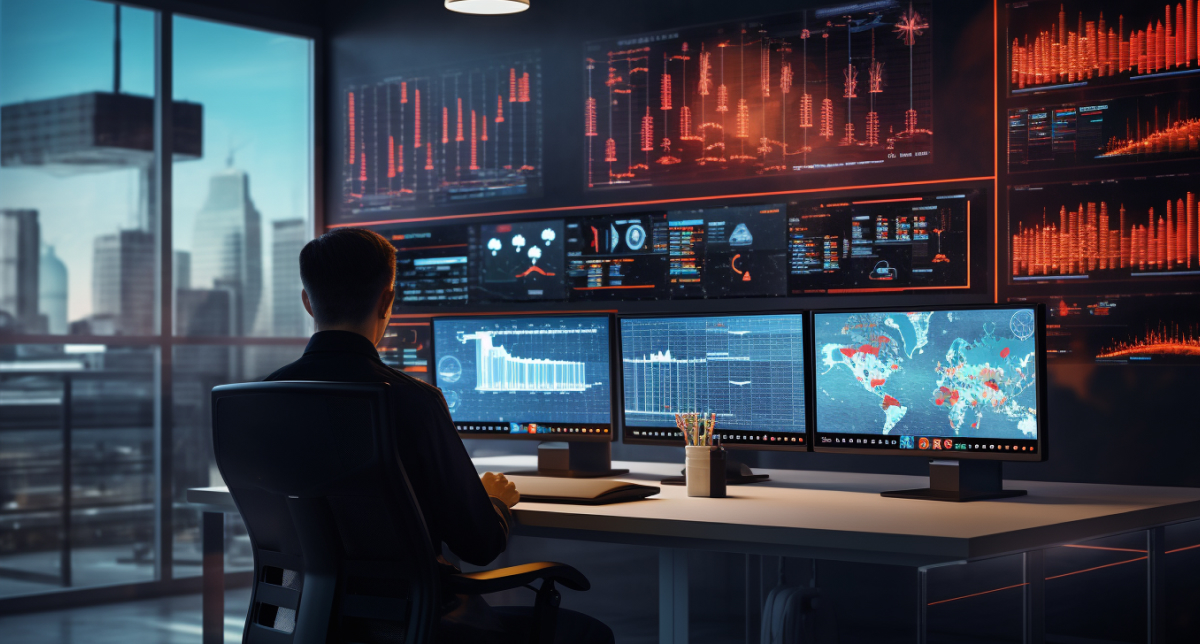
Automation monitoring is crucial for businesses that rely heavily on automated systems. These systems are designed to increase efficiency and reduce costs. However, they can also cause significant issues if not appropriately monitored. For example, if an automated system that manages inventory does not solve its RPA challenge, a business could run out of stock, causing a loss of sales and revenue. Similarly, if an automated system responsible for processing orders fails, it could lead to delayed shipments and dissatisfied customers.
How Can Machine Learning Be Used For Automation Monitoring?
Machine learning can be used for automation monitoring in a variety of ways. Here are some examples:
Predictive Maintenance
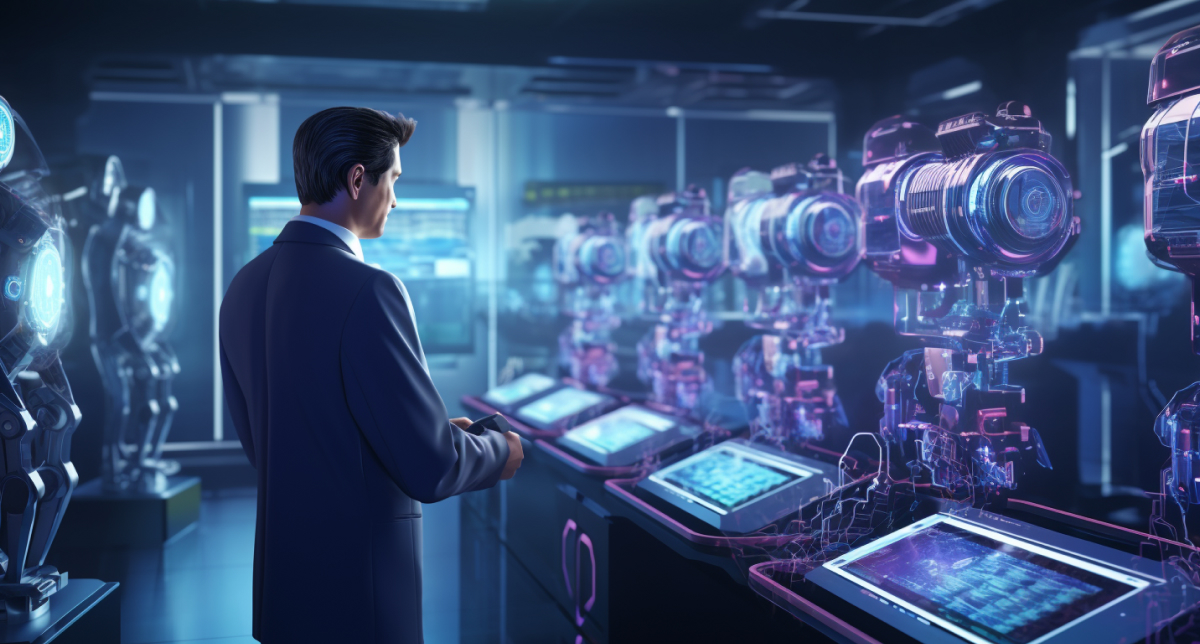
RPA as a service can predict when a piece of equipment is likely to fail, based on historical data. This allows businesses to schedule maintenance before a breakdown occurs, minimizing downtime and reducing costs.
Anomaly Detection
RPA implementation can also detect anomalies in data, such as unusual spikes or dips, which may indicate a potential issue. This allows businesses to address the issue before it causes any problems.
Process Optimization:
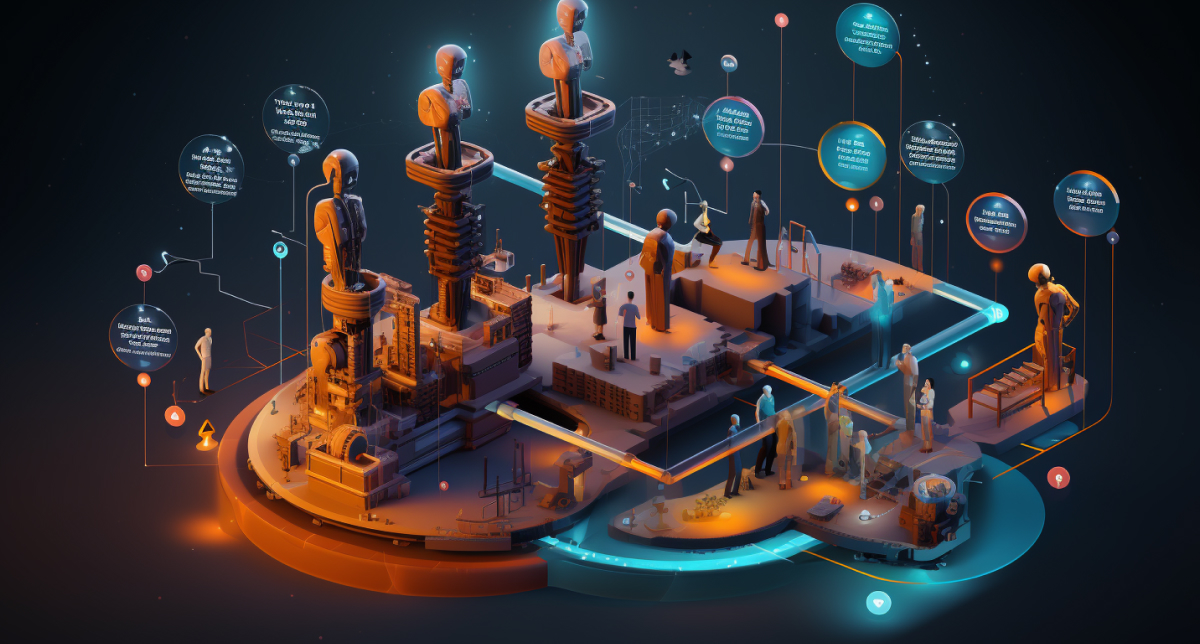
RPA as a service can identify room for improvement, allowing businesses to optimize their processes and improve efficiency.
Root Cause Analysis:
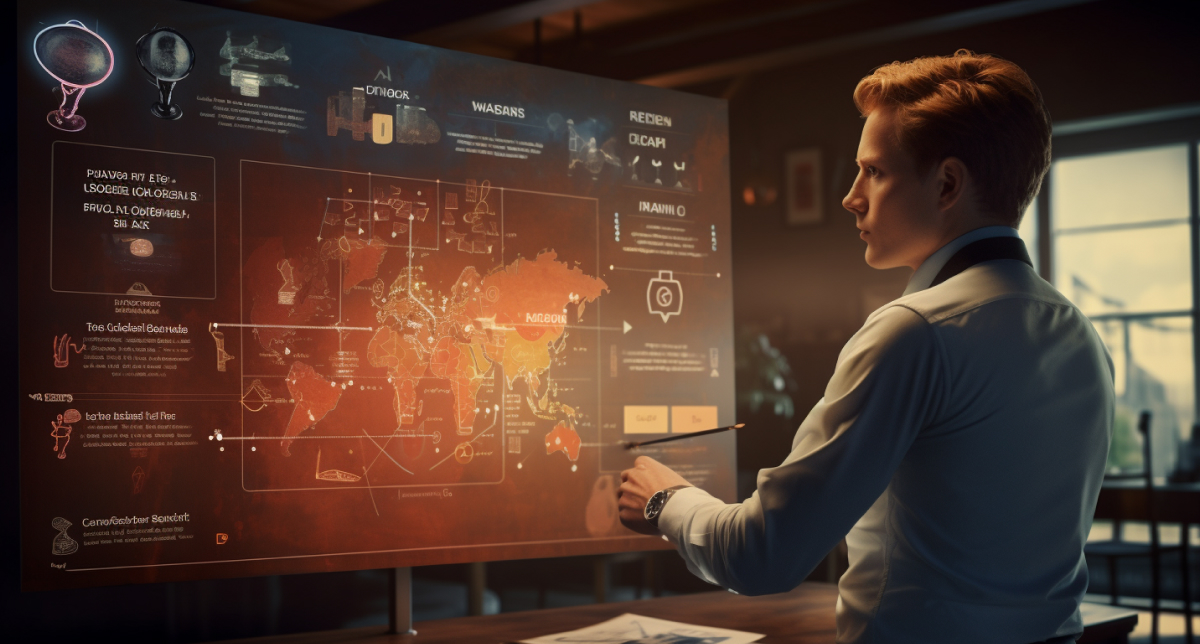
Machine learning algorithms can be devised to avoid issues that follow the same pattern. This can help to prevent them before they occur again.
What Are The Benefits Of Using Machine Learning For Automation Monitoring?
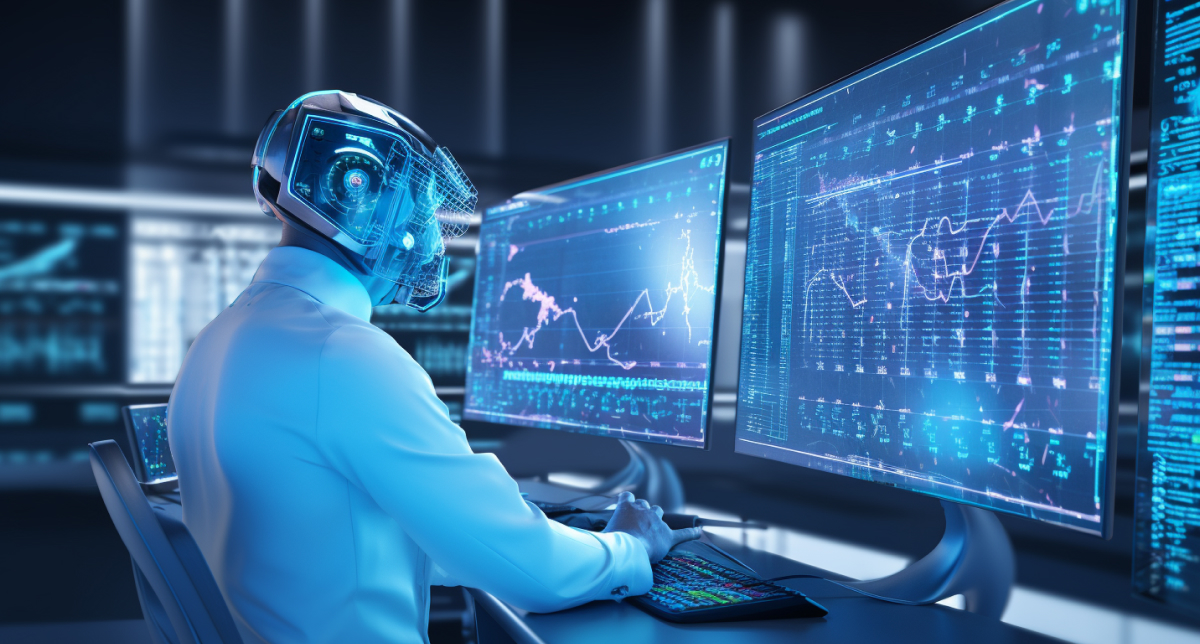
The benefits of using machine learning for automation monitoring are numerous. Here are a few examples:
- Improved Efficiency: Machine learning algorithms can optimize automated systems, increasing efficiency and reducing costs.
- Reduced Downtime: Predictive maintenance can help businesses schedule maintenance before a breakdown, minimizing downtime.
- Improved Customer Satisfaction: By addressing issues before they occur, businesses can ensure that their customers receive their orders on time and in good condition, leading to higher customer satisfaction.
- Cost Savings: By preventing issues beforehand, businesses can save money on repairs and replacements.
Conclusion
Machine Learning is on its course to change how a business approaches automation monitoring. By using predictive maintenance, anomaly detection, process optimization, and root cause analysis, businesses can proactively address issues before they occur.
The benefits of using machine Learning for automation are similar to process automation—increased efficiency, reduced downtime, technological advancements etc. In the coming times, machine learning is highly likely to integrate better with the automation monitoring process, helping businesses stay ahead of the curve and remain competitive in their respective industries. The RPA challenge is not very hard to crack, but a rigorous approach to problem-solving is needed.